The Emergence of Shadow AI and Why Evolution, Not Revolution, Might Just Kill it Dead
Published 01/31/2024
Originally published by CXO REvolutionaries.
Written by Martyn Ditchburn, CTO in Residence, Zscaler.
Cyber professionals are being bludgeoned daily by the topic of AI from both within their organizations and without. As a colleague acknowledged in a recent roundtable – the largest abuse of data in history is currently underway and it involves AI. He was referring to the use of intellectual property in Hollywood and content producers’ ongoing efforts to stem the use of AI-generated content.
Given this turbulence, it is hard to believe that we are living through anything short of an AI revolution. But it does not necessarily follow that cyber professionals’ response must be similarly momentous. The solution to GenAI risk is, in my opinion, far simpler and will resemble evolution more than revolution.
I recently had the opportunity to discuss AI/ML-related fears with a group of industry-leading CISOs, focusing on how we might arrive at an acceptable level of risk associated with its use. A significant portion of the group believed there were not adequate tools available in the market to safely control AI and large language models (LLMs). As a result, their organizations had instituted a blanket moratorium on their use. What was left to fear?
In short, humans’ unpredictability and tendency toward user error.
Not only that, but GenAI became accessible to the layman almost overnight. Many CISOs issued the ban to prevent potential data leakage. One CISO told me he simply did not have the budget or the time to explore user education campaigns. Phishing has been around since the late 1990s and how many users still take the bait each day? He simply felt that leaving GenAI use up to the user’s discretion would be a misstep. An outright ban seemed sensible for putting the genie back into the bottle, at least until his organisation had time and resources to deal with it comprehensively.
But wait. Hasn’t our industry encountered this issue before? I am of course referring to the birth of Shadow IT and the earliest days of cloud data storage and processing. CISOs and IT professionals (myself included) outlawed cloud usage. We told ourselves then that this was only temporary until we had time to think about it properly (we never did until we were forced to). I am reminded of how, some ten years ago, I believed my organisation would never be risk-tolerant enough to embrace the cloud. Yet, just five years later, we were all in. Let us not kid ourselves, however, as to why this cloud revolution happened in the first place: it was driven by the desire for a competitive advantage. Shadow IT showed us what happens when you simply say “no.”
Does this mean we all must strap in to deal with the emergence of Shadow AI? The group reached a consensus pretty quickly that we would not want that brain-vomit term to go mainstream. So, how could we stop Shadow AI from becoming a thing?
"The definition of insanity is doing the same thing over and over and expecting different results." – Albert Einstein
Let us take a leaf out of our own IT history book and learn from the failings that gave birth to Shadow IT. To do that, we must embrace AI. Not only that, we have to use it as a chance to gain a competitive advantage over slower-moving rivals.
But before we unleash the world of AI onto our users, let us first take a moment to consider the areas we might want to restrict, permit, and monitor.
Restrict inbound
Not all LLMS are created equal. Public data versus private data inputs matter. Public data is at best unregulated and at worst it is biased, fake, or potentially dangerous. If you were to point GenAI at the world's Instagram collective, for example, it would quickly conclude that we all spend our lives on holiday. Conclusions from public sources cannot be trusted; the data’s foundation is flawed and highly selective. Models trained on these sources and only these sources, therefore, should be treated with skepticism. Making business decisions based on output from this type of LLM is not prudent.
Restrict outbound
A lack of awareness of AI’s hazards, in particular the speed at which it can ingest data, is not well understood among users. As such, horror stories are emerging in the form of data leakage. Just recently, I learned of a user who installed a plugin on their desktop allowing a public AI to scan non-confidential data. The user did not understand that any sub-directory was also in scope and therefore inadvertently shared far more than intended. This is a common form of data leakage and one CISOs know all too well.
An example of the “evolution over revolution” concept I introduced earlier would entail using existing DLP capabilities to prevent leakage from the endpoint. The group also recognised that the speed at which AI could ingest data presents an increased threat to the level of risk relating to unstructured data. As such, we recommended doubling down on DLP and data classification of unstructured data whenever possible.
Permit private AI instances
This is the crucial tactic for killing Shadow AI dead in its tracks. Simply put, make private LLM and AI services available to users, or at least a privileged group. This will channel energies toward an instance that can be monitored and controlled. In my experience, strong guardrails are more effective than blanket bans over the long term. Private data ingestion could be detected and reversed as necessary or allowed within the bounds of acceptable use policies.
Evaluate
Simply allowing or blocking AI-enabled LLMs is a technical response to what is ultimately a legal and ethical challenge. Work within your organisation to establish a code of conduct and ethical foundation to be sure it's permitted when possible and curtailed when necessary.
Current AI legislation is limited at best and will take years if not decades to be fully ironed out, so anticipate the need for self-regulation. Work towards an ethical and sustainable AI use policy, much like organisations had to do to satisfy environmental compliance measures.
Ultimately, my group of peers could not come to a conclusion regarding the ethics or practicality of using AI/ML to accelerate the categorisation of unstructured data. All could agree, however, that data classification is a core concern facing security professionals today and requires much more investment. After all, how can we protect what we do not know we have?
Whatever you decide is right for your organisation, it is clear that the capabilities to block, permit, and evaluate already exist in organisations' security toolkits. Whilst AI may be revolutionary, our response as security professionals should aim for something more evolutionary in its approach.
Related Resources
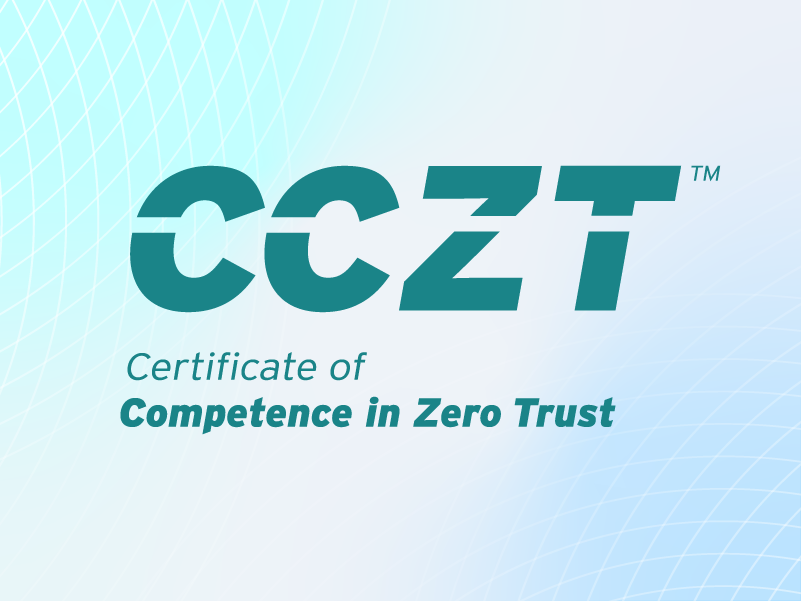
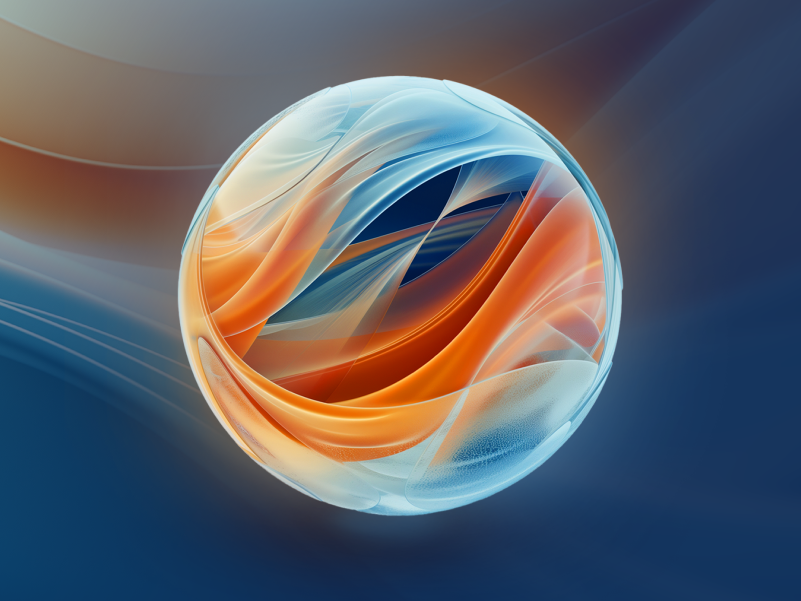
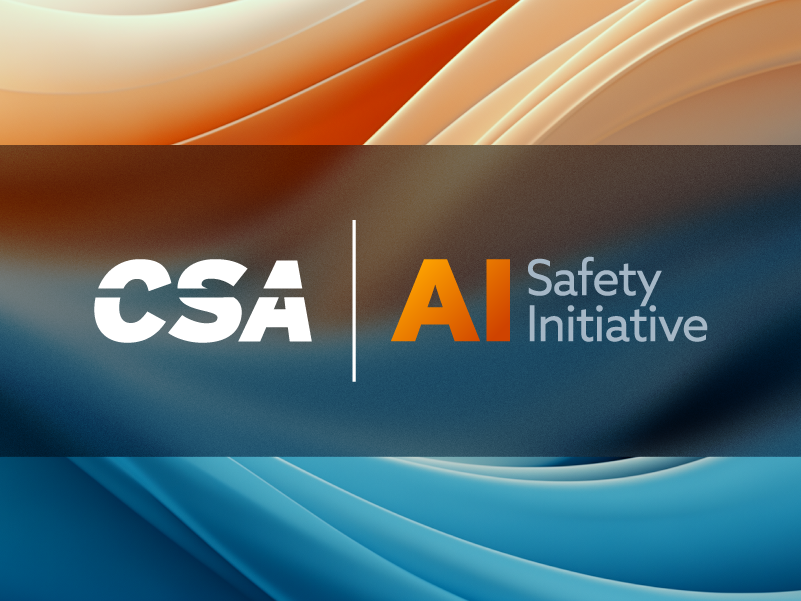
Unlock Cloud Security Insights
Subscribe to our newsletter for the latest expert trends and updates
Related Articles:
In the Beginning, Before Zero Trust
Published: 06/06/2025
Make Tech Changes Fun for End Users and Off-the-Chart Adoption Will Follow
Published: 06/06/2025
When Good GPTs Go Bad: How Trusted AI Tools Are Exploited for Attacks
Published: 06/05/2025
The Dawn of the Fractional Chief AI Safety Officer
Published: 06/04/2025