Beyond the Black Box: How XAI is Building Confidence
Blog Article Published: 03/28/2024
Written by Dr. Chantal Spleiss, Co-Chair for the CSA AI Governance & Compliance Working Group.
While "AI" has become a broadly used word, there are key distinctions within AI to keep in mind. Narrow AI systems excel at specific tasks, like playing chess or recognizing objects in images. Generative AI (GenAI) is a rapidly growing field that involves creating new text, images, code, or other forms of content. These systems pose unique challenges for understanding their output and ensuring it is not just logical but also correct. General AI, the ability to think and reason like a human across any domain, is still a distant goal.
Most real-world AI applications today fall into the categories of machine learning (ML) and deep learning. Machine learning uses algorithms to find patterns in vast amounts of data without being explicitly programmed. Deep learning employs layered "neurons" inspired by the structure of the brain, making them even more adept at handling complex, unstructured data.
The impressive abilities of these AI systems come with a caveat: it can be difficult to understand exactly how they arrive at their decisions. This "black box" problem raises concerns about reliability, especially when AI is used in high-stakes situations like medical diagnosis or loan approvals.
That's where Explainable AI (XAI) comes in. It's a field dedicated to developing techniques that help us understand the reasoning behind an AI's output. This is crucial not only for identifying potential mistakes but also for ensuring that those who use and are affected by AI systems can understand and, if necessary, challenge the results. While perhaps not the fastest-growing field, XAI is one of the most important. Here are a couple of examples demonstrating the shift from AI to XAI, which allows not only explaining results but also taking corrective actions:
Example 1: Healthcare Diagnosis
- Problem: Using medical imaging, an AI system helps identify heart issues in newborns. However, the AI system cannot explain why it classifies a particular patient as being at risk. This lack of transparency makes doctors hesitant to fully trust its recommendations.
- XAI Solution: Techniques that highlight the specific areas of the image the AI focuses on when making its diagnosis. This helps doctors verify if the AI is paying attention to the correct features, building confidence in the system's accuracy and reliability.
Example 2: Self-Driving Cars
- Problem: A self-driving car is involved in an accident. Investigators need to determine why the car made the decisions it did to assign liability (driver error, software glitch, sensor failure, etc.).
- XAI's potential Solution: XAI techniques hold the promise of reconstructing what the AI "saw" through its sensors and how it processed that information leading up to the crash. Such insights could address safety flaws and hold the appropriate parties accountable but are still under development.
This shift from focusing solely on AI's performance to understanding and correcting its output allows for the integration of CAPA (Corrective and Preventive Actions), facilitating a useful, iterative quality management process for AI. Holistically applied, iterative (continuous) improvement can better performance as well as resilience. While performance is the main focus in a revenue-driven market, resilience is often overlooked, expensive, and from a legal standpoint, hard to regulate. To balance a performance-driven market, accountability and liability are shifting into the picture. The recent offering for “hallucination insurance” highlights the shift towards increased (financial) liability in the AI field. Laws like NIS2 (Europe) and SEC (mainly the USA) underscore this shift in the level of cybersecurity by explicitly assigning accountability, responsibility, and liability for incidents to company management.
Make Safety a Priority, or Pay Twice the Price.
Related Resources
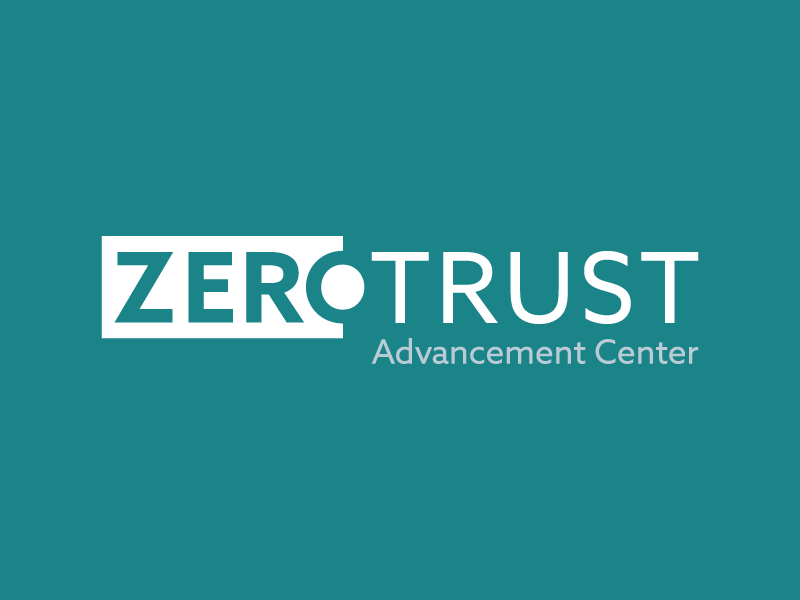
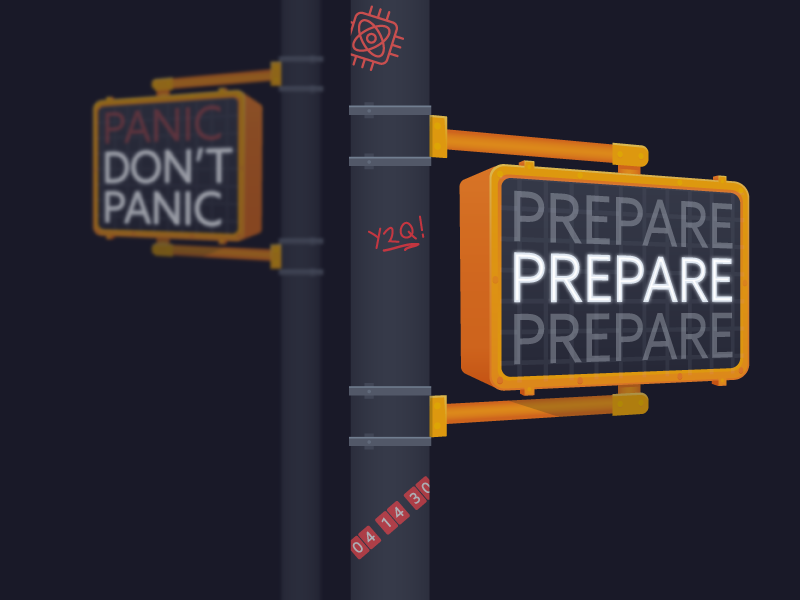
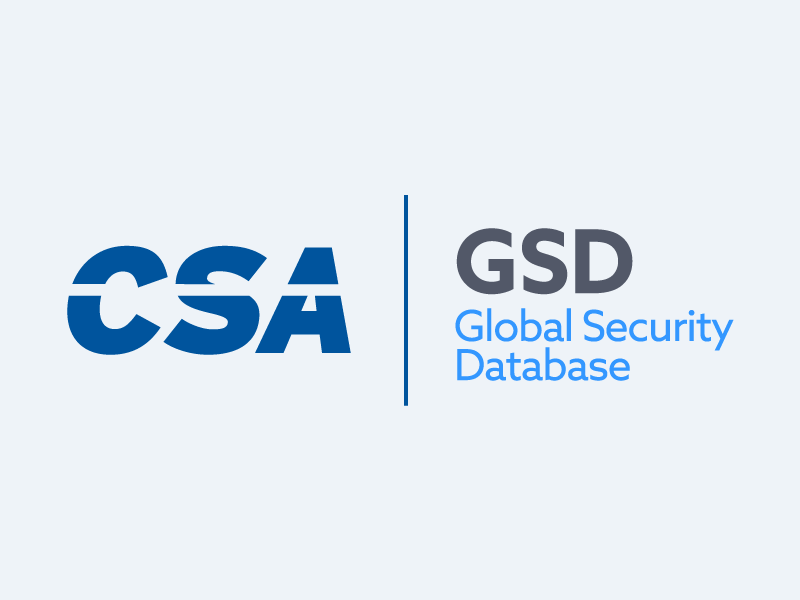
Related Articles:
CISOs, AI, and OT: A Balancing Act Between Innovation and Protection
Published: 05/03/2024
Microsoft Copilot for Security: Everything You Need to Know
Published: 05/02/2024