AI Data Considerations and How ISO 42001—and ISO 9001—Can Help
Published 06/28/2024
Originally published by Schellman.
While the rapid pace at which artificial intelligence (AI) technology has been both developing and impacting several areas of our daily lives continues, so too do the concerns about the tech’s safety, privacy, and bias. As there’s no stopping the ongoing integration of AI, organizations are now wondering what they can do to ease those concerns, and the answer is simple—start with protecting your data.
“Closing the box” on AI won’t happen, but everyone likely agrees that guardrails do need to be put into place. Together, we need to ensure that this powerful and disruptive technology has the right level of oversight so it doesn’t cause any undue harm to individuals, groups, or society as a whole as advanced innovation of this technology continues—everything hinges on AI’s responsible management.
Thankfully, there have been recent developments in AI risk management guidance and requirements, but you still might be wondering what to focus on / prioritize first when it comes to establishing safeguards and guardrails within your organization.
That’s where we can help. In this article, we’ll break down A.7 in Annex A of ISO 42001—which deals with data considerations in AI systems—as well as how ISO 9001 certification may be a helpful backdoor into getting your AI data into a trustworthy place so that you can get started in the way that works best for you.
Getting Started with Prioritizing Your AI Data
It’ll come as no shock, but data is central to AI systems. Remember the adage “garbage in, garbage out?” It applies very well in this context because AI is not inherently “good” or “evil,” “fair” or “biased,” “ethical” or “unethical”–whether it becomes one or the other of those dichotomies starts with the data—if bad data is used to build and train AI models, those models will inherently yield poor / unreliable results.
So how can creators and providers of AI technology avoid that? Where should you start in implementing guardrails that can foster trust and confidence in your products and services? Because while AI technology has been around for quite some time in one form or another, the concept of AI governance, risk, and compliance is fairly new, in terms of having established regulations—such as the EU AI Act—that can help build trust.
Still, a few risk-based frameworks and standards have been recently introduced—including the NIST AI Risk Management Framework (AI RMF) and ISO 42001. Both share the goal of helping organizations responsibly utilize AI technology through a risk-focused approach to the design, development, and/or utilization of AI within their organizations and in their product and service offerings.
A.7 in Annex A of ISO 42001: A Breakdown
To learn more about either, you can check our articles on the AI RMF and ISO 42001, but going forward, we’ll focus on the latter and its criteria for building out an AI management system (AIMS)—specifically, the A.7 control objective in Annex A of ISO 42001 which breaks down like so:
A.7 Data for AI Systems |
||
Objective: To ensure that the organization understands the role and impacts of data in AI systems in the application and development, provision or use of AI systems throughout their life cycles. |
||
Topic |
Control |
|
A.7.2 |
Data for development and enhancement of AI system |
The organization shall define, document and implement data management processes related to the development of AI systems |
A.7.3 |
Acquisition of data |
The organization shall determine and document details about the acquisition and selection of the data used in AI systems. |
A.7.4 |
Quality of data for AI systems |
The organization shall define and document requirements for data quality and ensure that data used to develop and operate the AI system meet those requirements. |
A.7.5 |
Data provenance |
The organization shall define and document a process for recording the provenance of data used in its AI systems over the life cycles of the data and the AI system. |
A.7.6 |
Data preparation |
The organization shall define and document its criteria for selection data preparations and the data preparation methods to be used. |
While this isn’t the first control objective in Annex A of the ISO 42001 standard, you can see that it lays out the sequential considerations of data well, making it a crucial starting point when performing an AI risk analysis within any organization.
Key Considerations for Data in AI Systems (Per A.7 in Annex A of ISO 42001)
However, a sequence to follow regarding your data isn’t enough to build trust in your AI systems, so here’s a summary of the controls and key considerations to make when implementing safeguards for your AI data:
Subsection | Where to Start |
A.7.2 Data for development and enhancement of AI system |
|
A.7.3 Acquisition of data |
|
A.7.4 Quality of data for AI systems |
|
A.7.5 Data provenance |
|
A.7.6 Data Preparation |
|
The Data Benefits of Integrating ISO 42001 and ISO 9001
When ISO 42001 was introduced at the end of 2023, it was immediately apparent—through several references to ISO 27001, ISO 27701, and ISO 9001—that it was drafted in a way to integrate well with these other management system standards, but it’s the link to that last standard that we find could be very valuable.
Why?
With AI, folks often only consider the security and privacy implications—which is where ISO 27001 and ISO 27701 certifications naturally come in. And while those shouldn’t be ignored in their own right, the more AI-specific issues like bias and the related unintended consequences / negative outcomes are often instead the result of poor data management, and that’s where ISO 9001 can help.
See, AI models depend entirely on the data set they’re trained on—they’re fed information and reflect that in their outputs. It makes sense then, that bias in AI models is often a data representation issue (e.g., where healthcare systems leveraging AI are only trained on data from a certain sect of the population and thus yield naturally biased results towards that specific population). So to build trust in your AI systems, you’ll obviously need to address potential bias issues as they relate to how your models operate and make decisions. That means proving they’re centered around transparency, which can only happen if you have a firm grasp on:
- The source(s) of your data;
- How representative it is of the target user population; and
- How that data is continually trained over time to ensure that the AI models’ decisions / output remain reliable.
Here’s where ISO 9001 comes in.
That standard’s goal is facilitating business across organizations and inspiring customer confidence in products and services through the implementation of a quality management system (QMS). Being so designed to promote customer confidence in the quality of reliability of products and services—something that is highly data- and process-driven—ISO 9001 makes a great complement to ISO 42001, as it can provide even more specific guidelines in data-related areas like risk management, software development, and supply chain coherence, etc.
Therefore, when an AIMS is implemented jointly with a QMS, customer confidence can be even further reinforced—so it may benefit your organization greatly to do so.
Next Steps
Artificial intelligence and its ongoing assimilation into society are both exciting and concerning at the same time. While everyone is certainly happy to see daily tasks become more automated and efficient with the predictability and prevention that AI offers—e.g., your GPS predicts traffic patterns to avoid an accident and reroutes you automatically to get to your final destination quicker—consumers also want to know that those systems can be trusted, and that starts with good data.
The ISO 42001 standard can help organizations prove their overall responsibility in managing AI, but A.7 in Annex A within the framework is also a great place to start when implementing guardrails around the data within your AI models. Now that you know a little more about it—as well as specific data considerations to make—you may also want to consider reviewing the ISO 22989 standard, which contains more detailed information on AI system lifecycle and data management concepts.
Related Resources
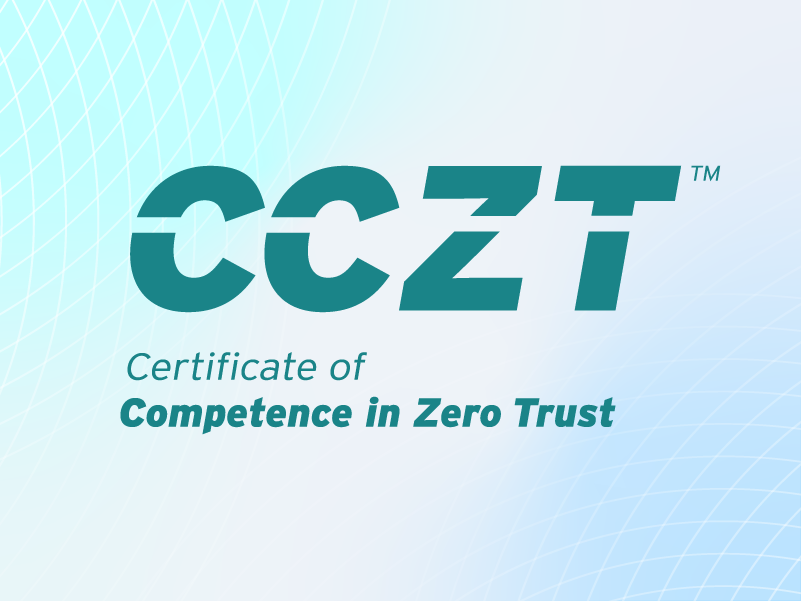
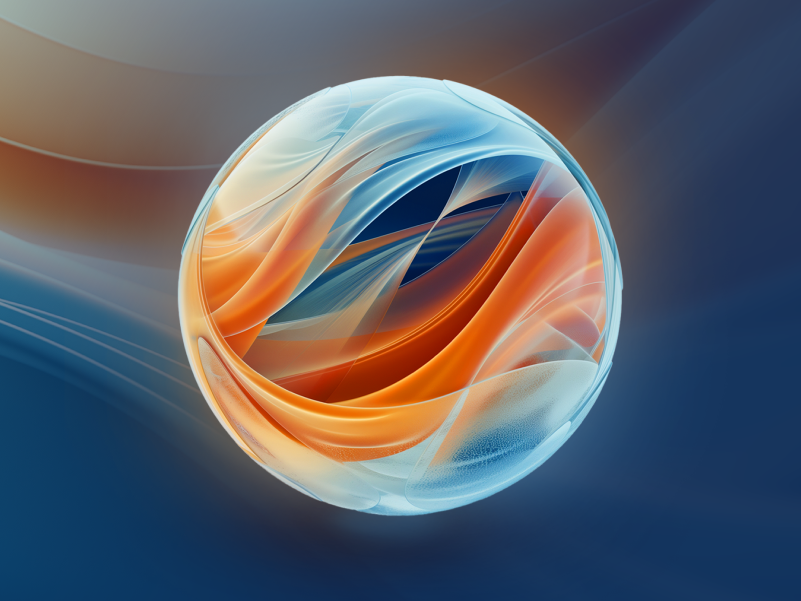
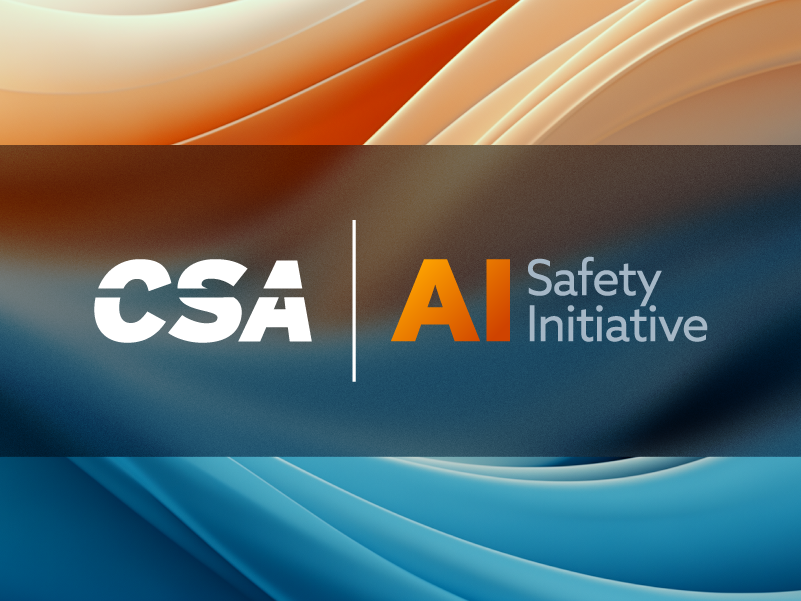
Unlock Cloud Security Insights
Subscribe to our newsletter for the latest expert trends and updates
Related Articles:
Policy-as-Code vs. IaC Security: What’s the Real Difference?
Published: 07/08/2025
Why Identity Automation Fails at 96% of Organizations
Published: 07/07/2025
6 Key Steps to ISO 42001 Certification Explained
Published: 07/07/2025
What MITRE ATT&CK v17 Means for ESXi Security: Key Risks & How to Respond
Published: 07/03/2025