Next-Gen Cybersecurity with AI: Reshaping Digital Defense
Published 01/10/2025
Written by Rahul Kalva.
Abstract
As the cyber threat landscape evolves, leveraging Artificial Intelligence (AI) has become imperative for creating robust, proactive defenses. AI's ability to detect, predict, and respond to threats in real-time sets it apart as a transformative force in cybersecurity. This article explores AI-driven cybersecurity advancements, focusing on predictive analytics, automated responses, and adaptive defense systems, emphasizing their significance in safeguarding dynamic IT ecosystems.
Scope
This article examines the integration of AI into cybersecurity frameworks and its potential to mitigate evolving threats. We will discuss AI's role in threat detection, adaptive defense mechanisms, and its application in securing Internet of Things (IoT) networks. By addressing real-world applications and challenges, this article highlights how organizations can build resilient, AI-powered security strategies.
Introduction
The digital age has revolutionized how we interact, innovate, and operate businesses, but it has also introduced unprecedented cybersecurity challenges. Cybercriminals are employing sophisticated methods, such as AI-driven attacks, to exploit vulnerabilities. Traditional rule-based security measures often fail to address such complex threats. AI has emerged as a critical tool, enabling organizations to detect, respond to, and even predict cyber threats more effectively than ever before.
Defining AI in Cybersecurity
AI in cybersecurity involves the application of machine learning (ML), natural language processing (NLP), and other intelligent technologies to automate and enhance security processes. ML algorithms, for example, can analyze vast amounts of data to uncover unusual patterns and potential threats that would otherwise go unnoticed. These capabilities allow for more accurate and faster detection of security incidents, minimizing the time attackers must exploit vulnerabilities.
NLP plays a crucial role in understanding and analyzing vast amounts of unstructured data, such as email communication, chat logs, or threat intelligence feeds. By doing so, NLP enhances phishing detection, email filtering, and the identification of malicious intent in real-time, providing an additional layer of security against social engineering attacks.
Another powerful application of AI in cybersecurity is intelligent threat hunting. By automating the process of sifting through logs and system data, AI-driven tools can proactively identify potential risks and vulnerabilities. These tools not only improve the efficiency of security teams but also help to build a proactive security posture that adapts to evolving threat landscapes.
AI-Driven Threat Detection and Response
AI excels in processing vast datasets to identify patterns that signify malicious activity. Here are some pivotal aspects:
- Behavioral Analytics: AI creates a baseline for normal user behavior and flags deviations, such as unauthorized access or unusual data transfers.
- Predictive Threat Intelligence: By analyzing historical data and global threat vectors, AI can predict and preempt potential attacks.
- Real-Time Incident Response: AI-powered Security Information and Event Management (SIEM) systems automate responses to mitigate damage and contain threats.
Securing IoT Ecosystems with AI
The rise of IoT devices has exponentially expanded the attack surface for cybercriminals. AI addresses these vulnerabilities by:
- Device Profiling: Identifying and monitoring IoT devices for anomalous behavior.
- Edge AI Deployment: Ensuring rapid threat detection and response at the network edge.
- Automated Patch Management: Detecting vulnerabilities and deploying updates without manual intervention.
Adaptive Security Models
AI-powered adaptive security models evolve with the threat landscape:
- Self-Healing Systems: Automating the remediation of detected vulnerabilities in real time.
- Dynamic Risk Assessment: Continuously assessing and adjusting risk scores to prioritize security efforts.
Challenges in AI-Driven Cybersecurity
- Adversarial Attacks: Cybercriminals are leveraging AI to develop advanced
- evasion techniques.
- Data Privacy Concerns: Striking a balance between data analysis and privacy is critical.
- Resource Intensive: Implementing AI solutions can require significant investment in
- infrastructure and expertise.
Recommendations for Implementation
To maximize the potential of AI in cybersecurity, organizations should:
- Adopt Hybrid Models: Combine AI with human expertise for comprehensive threat analysis.
- Invest in Training: Upskill security teams to manage AI tools effectively.
- Foster Collaboration: Engage with industry partners to share threat intelligence and best practices.
Conclusion
The integration of AI into cybersecurity is redefining how organizations defend against threats. By automating detection, enhancing incident response, and enabling predictive intelligence, AI offers a proactive approach to safeguarding digital assets. As cyber threats grow in sophistication, embracing AI is no longer optional, but essential for building a resilient security framework.
About the Author
Rahul Kalva is a seasoned expert in DevSecOps, cloud architecture, and AI, with over 20 years of experience shaping secure, scalable enterprise technology solutions. Known for his leadership and technical acumen, Rahul has made significant contributions to the field, focusing on advanced security practices and cloud innovation. He is also a dedicated member of the Cloud Security Alliance SFO Chapter, where he collaborates with industry peers to advance cloud security practices.
Rahul holds professional certifications across major cloud platforms, including AWS, Azure, and GCP, along with extensive expertise in Kubernetes, Terraform, and containerization, which further solidify his ability to build resilient, high-performance systems. His work spans various industries, where he has architected secure, automated DevOps pipelines and implemented AI-driven solutions to enhance operational efficiency and security.
With a deep commitment to advancing security in cloud-native and hybrid environments, Rahul excels in integrating cutting-edge DevSecOps methodologies with AI for proactive threat detection and response. His approach emphasizes customer-focused solutions that align technology with strategic business goals, delivering secure, innovative outcomes that drive value across organizations.
Related Resources
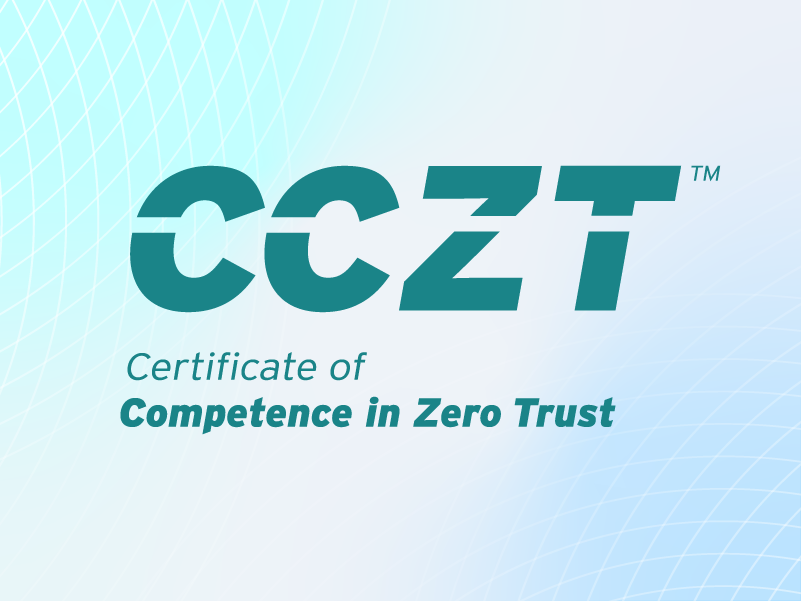
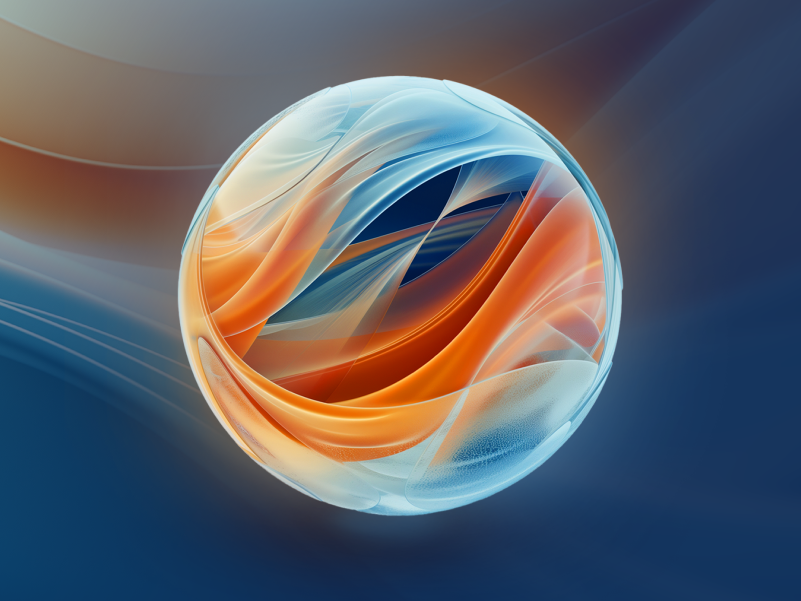
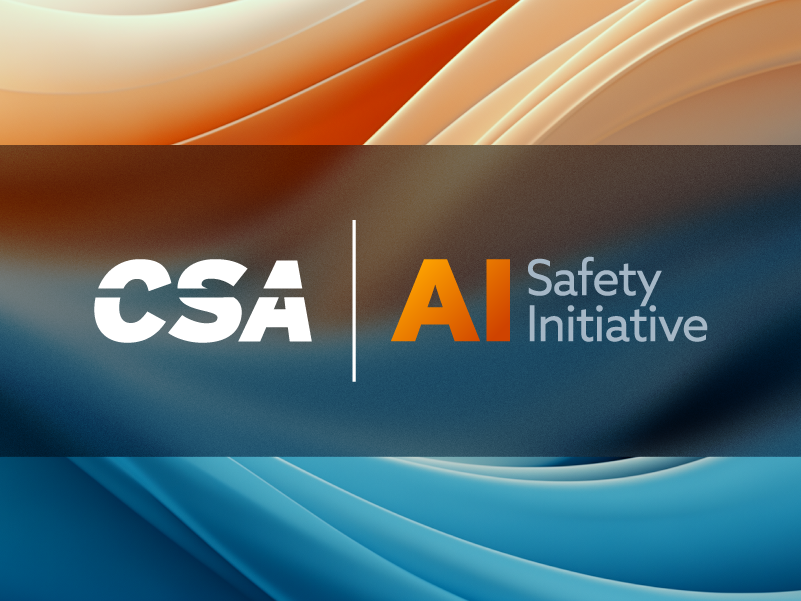
Unlock Cloud Security Insights
Subscribe to our newsletter for the latest expert trends and updates
Related Articles:
Roadmap to Agentic AI Implementation
Published: 06/02/2025
A Global Snapshot of AI Laws and How Compliance with ISO 42001 Can Help
Published: 06/02/2025
AI Cybersecurity Regulations: What CISOs Need to Know
Published: 05/30/2025
The True Costs of Legacy PAM: What an Outdated System is Really Costing You
Published: 05/29/2025