Data-Driven AI: Understanding the Risks and Mitigations
Blog Article Published: 08/15/2023
Written by Tarun Gupta, Enterprise Risk, Compliance and Assurance Leader, G42.
In recent years, the rapid advancements in artificial intelligence (AI) have revolutionized various industries, unleashing unprecedented opportunities for innovation and growth. Among the various approaches to AI, "Data-Driven AI" stands as a powerful paradigm that relies on large volumes of data to enable machines to learn and make intelligent decisions. By extracting patterns and insights from massive datasets, Data-Driven AI has proven itself indispensable in areas such as finance, healthcare, transportation, and beyond.
While the potential of Data-Driven AI is vast, it also brings along a suite of challenges and risks that demand careful consideration. Understanding these risks and devising appropriate mitigations is of paramount importance for responsible AI implementation and ensuring its ethical and sustainable usage.
This article delves into the critical topic of Data-Driven AI, aiming to explore the risks that arise from its data-centric nature and the strategies employed to mitigate those risks. We will examine how the ever-increasing volume and diversity of data can impact AI systems, affecting their performance, fairness, and overall reliability. Additionally, we will shed light on the ethical concerns associated with the collection, storage, and usage of vast amounts of personal and sensitive information.
Risk 1: Missing Data Verification and Data Pipeline Replication
One of the critical challenges in AI security is ensuring the accuracy and standardization of data sourcing. Missing data verification and data pipeline replication can contribute to a lack of standardization, potentially leading to severe consequences for AI models. Verification of data involves confirming the presence, accuracy, and completeness of data points within a dataset, while data pipeline replication ensures consistency and reliability in data processing.
When data verification is not properly conducted, erroneous or incomplete data may enter the AI training process, leading to biased models and inaccurate predictions. Furthermore, data pipeline replication ensures that the data processing steps are consistent across different environments and systems. Failing to replicate data pipelines correctly can result in inconsistencies in data preprocessing and feature extraction, leading to unpredictable model behavior.
To mitigate these risks, organizations must establish robust data verification procedures, which include data quality checks, validation techniques, and data cleaning methods. Additionally, implementing automated data pipeline replication mechanisms helps maintain data consistency throughout various stages of the AI development process.
Controls to Mitigate:
- Data Governance and Compliance (DG): Establish robust data verification procedures that include data quality checks, validation techniques, and data cleaning methods to ensure the accuracy and completeness of data points within datasets.
- Information Security (IS): Utilize encryption and access controls to protect data during the verification and replication processes, preventing unauthorized access and tampering attempts.
- Risk Management and Assessment (RMA): Conduct regular risk assessments to identify vulnerabilities in data pipelines and verification processes. Develop risk mitigation strategies to address identified risks and improve data integrity.
Risk 2: Vendor Risks and Data Pedigree
Vendor risks pose significant challenges to AI security, particularly regarding the pedigree of data. Organizations often rely on external vendors for data sourcing, and without proper due diligence, they may inadvertently incorporate inaccurate or malicious data into their AI systems. A thorough assessment of the data sources' components, inputs, systems, and processes is crucial to understanding the historical context and potential biases associated with the data.
By understanding the pedigree of the data, organizations can better identify potential vulnerabilities and take appropriate measures to address them. This may involve conducting audits of the vendor's data collection practices, ensuring data privacy compliance, and establishing clear data-sharing agreements to protect sensitive information.
Controls to Mitigate:
- Vendor Management (VM): Conduct thorough due diligence on data vendors to assess the pedigree of the data they provide, ensuring data quality and reliability.
- Data Privacy and Compliance (DPC): Establish clear data-sharing agreements that include data privacy and security requirements to protect sensitive information. Regularly audit data vendor compliance to safeguard data integrity.
Risk 3: Insufficiently Large and Comprehensive Data Sets
Data is the backbone of AI models, and insufficiently large or comprehensive datasets can create significant data risk. Inadequate data can lead to overfitting, where the model performs well on the training data but fails to generalize to new, unseen data. It can also lead to biased models, as the AI system may not have enough diverse examples to learn from.
To mitigate this risk, organizations must prioritize data collection efforts and invest in acquiring diverse and representative datasets. They should consider both quantity and quality of data, ensuring that the data sets used for training cover a wide range of scenarios and use cases relevant to the AI application. Data augmentation techniques can also be employed to increase the effective size of the dataset and enhance model performance.
Controls to Mitigate:
- Data Management and Encryption (DME): Prioritize data collection efforts and invest in acquiring diverse and representative datasets. Utilize data encryption techniques to protect sensitive data during data collection and storage.
- Data Governance and Compliance (DG): Implement data governance policies that define criteria for selecting comprehensive and representative training datasets. Regularly review data quality and completeness to identify and address data insufficiencies.
Risk 4: Limited Internal Availability of Data
AI systems often require large volumes of data to train effectively, and sometimes, the necessary data may not be available internally. Organizations might resort to acquiring data from external sources, which introduces additional security and privacy risks. When relying on external data, organizations need to be cautious about the quality, veracity, and potential bias in the acquired data.
To address this risk, organizations can explore collaboration opportunities with partners or institutions to access relevant datasets securely. Moreover, implementing proper data anonymization and data protection measures when dealing with external data sources is crucial to maintaining privacy and confidentiality.
Controls to Mitigate:
- Data Governance and Compliance (DG): Implement proper data anonymization and protection measures when dealing with external data sources to maintain privacy and confidentiality.
- Risk Management and Assessment (RMA): Conduct risk assessments to identify potential security and privacy risks associated with external data sources. Develop risk mitigation strategies to ensure secure handling of external data.
Risk 5: Inadequate Selection of Training and Testing Data Sets
The selection of training and testing data sets significantly impacts the performance and generalizability of AI models. Not fit-for-purpose selection of these data sets can lead to biased, overfitted, or unreliable models, posing serious security and operational risks.
To mitigate this risk, organizations should prioritize the careful curation of training and testing datasets. It is essential to ensure that these datasets are representative of the real-world scenarios the AI system will encounter. Employing cross-validation techniques and conducting thorough evaluation processes can help identify and address issues related to data selection.
Controls to Mitigate:
- Data Governance and Compliance (DG): Establish data governance policies that prioritize the careful curation of training and testing datasets. Define clear criteria for selecting representative datasets.
- Risk Management and Assessment (RMA): Identify and assess risks related to data set selection and its impact on model performance. Develop strategies to minimize the risk of biased or unreliable models.
Risk 6: Lack of Standardized Processes for Data Inconsistencies Detection and Remediation
The absence of standardized processes for detecting and remediating data inconsistencies can significantly impact the quality and reliability of AI models. Inconsistent data can introduce noise and bias, leading to inaccurate predictions and potentially harmful consequences.
Establishing standardized data quality control procedures is crucial to maintaining the integrity of AI models. This includes defining clear criteria for identifying and handling data inconsistencies, employing automated data validation and cleaning techniques, and conducting regular audits of the data pipelines to ensure consistency and accuracy.
Controls to Mitigate:
- Data Governance and Compliance (DG): Implement standardized data quality control procedures to detect and remediate data inconsistencies effectively.
- Risk Management and Assessment (RMA): Conduct risk assessments to identify potential data inconsistencies and their impact on AI model performance. Develop strategies to ensure accurate and reliable AI models.
Risk 7: Lack of Data Representativeness
Data representativeness is vital for training AI models that generalize well to diverse real-world scenarios. When certain elements are given lower or higher chances of being selected into the sample, or when some elements are entirely excluded (zero probability of selection), the data becomes non-representative and biased.
To address this risk, organizations should invest in rigorous data sampling methodologies that ensure each element has an appropriate chance of being included in the dataset. Techniques like stratified sampling can be used to maintain representativeness across different subgroups in the data.
Controls to Mitigate:
- Data Governance and Compliance (DG): Invest in rigorous data sampling methodologies, such as stratified sampling, to ensure data representativeness and avoid introducing biases.
- Risk Management and Assessment (RMA): Assess the risk of non-representative data and its impact on AI model performance. Develop strategies to ensure diverse and unbiased data representation in the training set.
Risk 8: Missing Data Normalization
Data normalization is a critical preprocessing step in AI training. When missing or improperly conducted, data normalization can lead to data redundancies, making the AI model more complex than necessary. Moreover, it can decrease data integrity and introduce inconsistencies during model inference.
To mitigate this risk, organizations should implement consistent data normalization techniques across their AI pipelines. Data scientists must be well-versed in normalization methods and their implications for the model's performance. Regular checks on data normalization and alignment with best practices can ensure the data remains accurate and efficiently processed.
Controls to Mitigate:
- Data Management and Encryption (DME): Implement consistent data normalization techniques across AI pipelines to maintain data accuracy and reliability.
- Risk Management and Assessment (RMA): Conduct risk assessments to identify potential data normalization issues and their impact on model performance. Develop strategies to mitigate risks related to data normalization.
Risk 9: Asynchrony and Asymmetry in Data Workflow Hand-offs
Asynchrony and asymmetry around data workflow hand-offs can create communication gaps within the development team. When team members have different levels of information or understanding about data processing steps and the AI model's workings, it can lead to miscommunication, errors, and security vulnerabilities.
To address this risk, organizations should foster a collaborative and transparent development culture. Regular team meetings, knowledge-sharing sessions, and documentation of data workflow processes can help bridge the information gaps and ensure that all team members are on the same page.
Controls to Mitigate:
- Communication and Information Sharing (CIS): Foster a collaborative and transparent development culture with regular team meetings and knowledge-sharing sessions to bridge information gaps.
- Risk Management and Assessment (RMA): Identify and assess risks related to communication gaps in the development team. Develop strategies to enhance information sharing and ensure all team members have adequate knowledge of data workflow processes.
Risk 10: Cloud-Native Attack Vectors and Permissions
The migration of AI workloads to public cloud environments introduces new security challenges. Cloud-native attack vectors may exploit vulnerabilities in cloud infrastructure, data storage, and communication channels. Additionally, permissions and access controls can become attack surfaces, allowing unauthorized access to sensitive data or AI models.
To mitigate cloud-specific risks, organizations must implement robust cloud security measures. This includes utilizing encryption for data at rest and in transit, configuring strict access controls based on the principle of least privilege, and regularly auditing and monitoring cloud resources for potential threats.
Controls to Mitigate:
- Cloud Security Management (CSM): Implement robust cloud security measures, including encryption, access controls, and continuous monitoring, to protect against cloud-native attack vectors and unauthorized access.
- Risk Management and Assessment (RMA): Conduct risk assessments to identify cloud-specific security risks and develop risk mitigation strategies to safeguard AI workloads in the cloud.
By understanding and implementing these top 10 data-related AI risks and their corresponding countermeasures, organizations can build resilient AI systems that inspire trust, deliver reliable outcomes, and propel the responsible and ethical deployment of artificial intelligence.
Related Resources
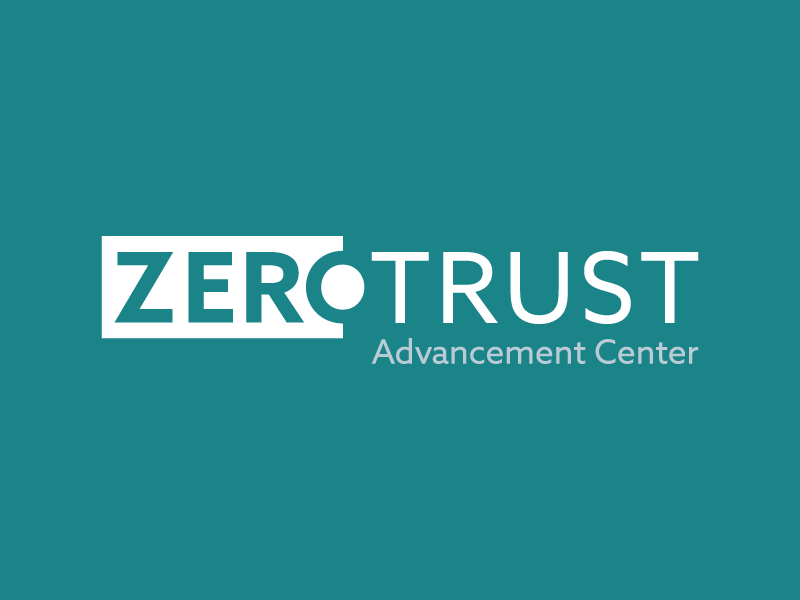
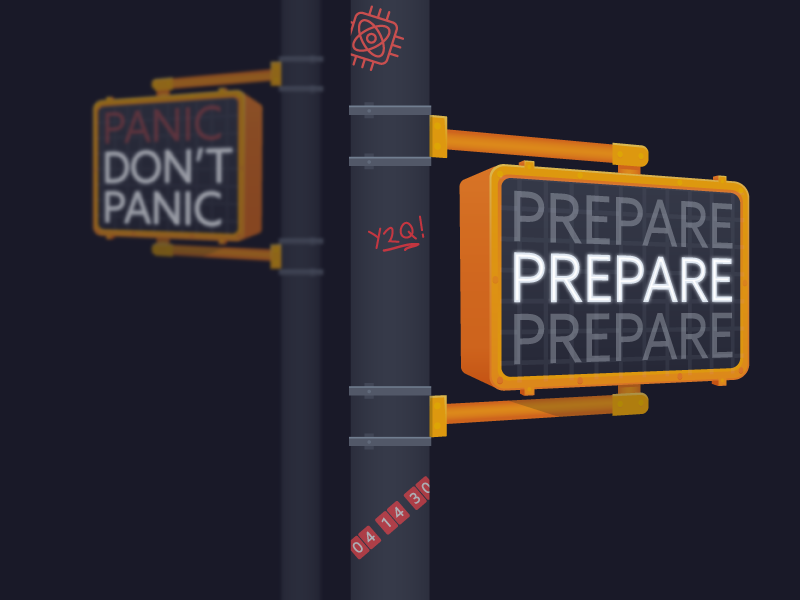
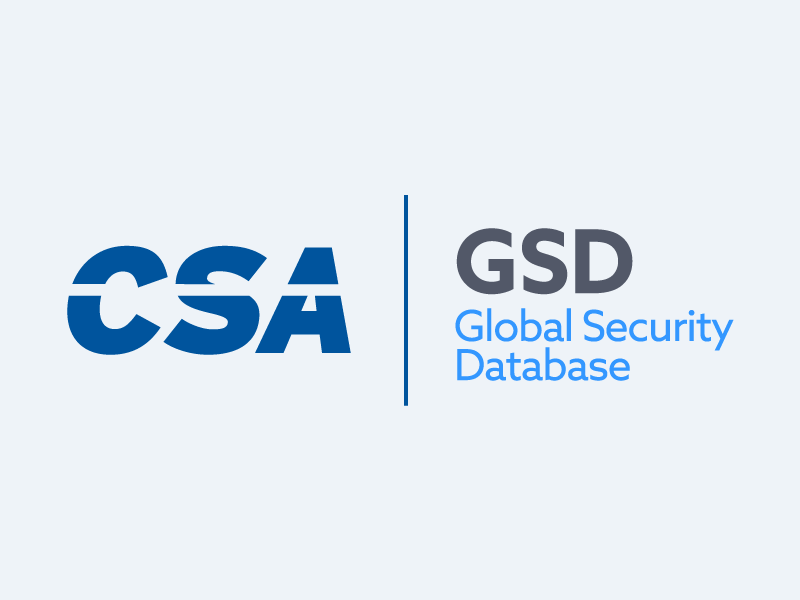
Related Articles:
The Risk and Impact of Unauthorized Access to Enterprise Environments
Published: 05/17/2024
Automated Cloud Remediation – Empty Hype, Viable Strategy, or Something in Between?
Published: 05/17/2024