Artificial Intelligence (AI) in Risk Assessment and Mitigation
Published 06/06/2024
Written by Ashwin Chaudhary, CEO, Accedere.
The advancement of generative AI technologies like GPT has led to rapid growth in AI adoption worldwide. While companies adopt AI with the intention of being competitive in the market, they often overlook the security risks that come with AI that can affect individuals, organizations, and the broader ecosystem. In this article, we’ll introduce you to the concept of AI risk management.
What is AI Risk Management?
A specialized branch of risk management, AI risk management is focused on identifying, evaluating, and managing the risks associated with the deployment and use of artificial intelligence.
This process includes developing strategies to address those risks to ensure the responsible use of AI systems that protect the organization, clients, and employees against adverse impacts from their AI initiatives.
AI Risk Management Framework
Several AI risk management frameworks have been introduced for more effective risk management. For example, the NIST’s AI Risk Management Framework a draft publication released on April 29, 2024, provides a structured way to assess and eliminate AI risks. It includes guidelines and best practices for using AI.
Risks Associated with AI
When discussing AI risk management, it is important to understand the risks that are associated with the use of AI. Risks can span domains such as security, privacy, fairness, and accountability. Here are a few common examples of risks for AI:
1. Data Privacy Risks:
- AI models, especially those trained on large datasets, can contain sensitive and personal information, such as Personally Identifiable Information (PII).
- These systems can inadvertently memorize and reveal sensitive information: this can result in an actual privacy breach and non-compliance with data protection regulations (like GDPR).
2. Bias in AI Models:
- Sometimes, the training data used to train AI models can include bias.
- It causes the AI model to produce inaccurate and discriminatory results.
3. Inaccurate Results:
- If the accuracy of the trained AI model is low, it can produce inaccurate results.
4. Overfitting:
- This phenomenon occurs when the AI model becomes too specialized for the training data.
- When new data is used, it can show poor performance.
Challenges and Examples
Some organizations have faced operational discrepancies due to inexperience with AI technology and a lack of clear frameworks. Few real-life instances:
1. Morgan Stanley restricted the use of ChatGPT by its staff due to concerns about hallucinatory outputs with factual inaccuracies.
2. Samsung banned its staff from using GenAI tools after sensitive intellectual property (IP) code was uploaded to such platforms.
3. Dutch “toeslagenaffaire” scandal involved incorrect penalization on citizens by the tax authorities for suspected childcare benefits fraud using a self-learning algorithm.
In a recent ‘Hype Cycle for Generative AI 2023 report’ by Gartner, by 2026, organizations that operationalize AI transparency, trust and security will see their AI models achieve a 50% improvement in terms of adoption, business goals and user acceptance.
How to Remediate the Associated AI Risks?
Conclusion
AI risk management is a crucial aspect of any organization’s AI strategy. By understanding and mitigating the risks associated with AI, organizations can ensure the responsible use of AI systems. As we move forward, it’s essential to stay updated with the latest developments in AI risk management to ensure the safe and effective use of AI.
It’s important to note that achieving high level of maturity in AI risk assessment and mitigation requires two to three years. Therefore, starting now is crucial.
About the Author
Ashwin Chaudhary is the CEO of Accedere, a Data Security, Privacy Audit, Technical Assessment and Training Firm. He is a CPA from Colorado, MBA, CITP, CISA, CISM, CGEIT, CRISC, CISSP, CDPSE, CCSK, PMP, ISO27001 LA, ITILv3 certified cybersecurity professional with about 22+ years of cybersecurity/privacy and 40+ years of industry experience. He has managed many cybersecurity projects covering SOC reporting, ISO audits, VAPT assessments, Privacy, IoT, Governance Risk, and Compliance.
Reference Links
- https://www.splunk.com/en_us/blog/learn/ai-risk-ma...
- https://www.onsolve.com/blog/the-future-is-now-ai-...
- https://risk-grid.com/portfolio/enhancing-risk-man...
- https://www.mdpi.com/2313-576X/10/2/42
- https://www.trinetix.com/insights/ai-in-risk-manag...
- https://www.nist.gov/itl/ai-risk-management-framew...
- https://securiti.ai/ai-risk-assessment/
Related Resources
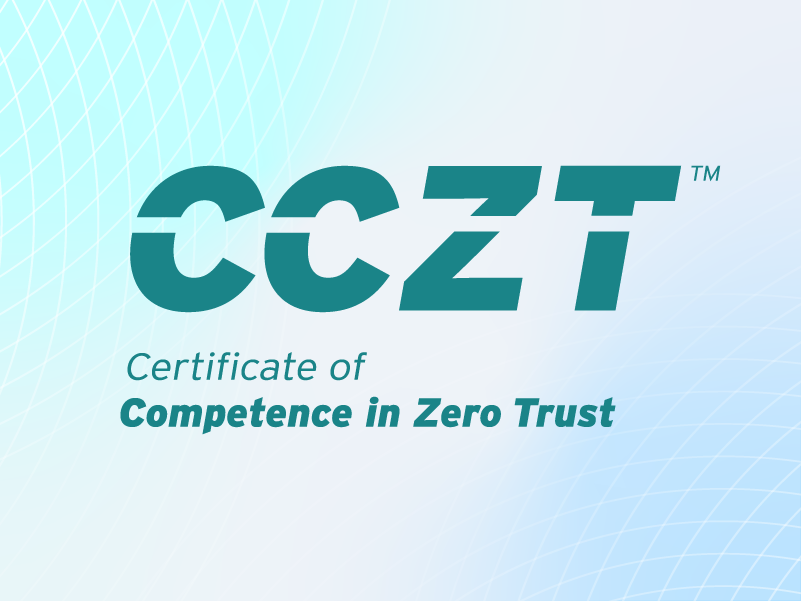
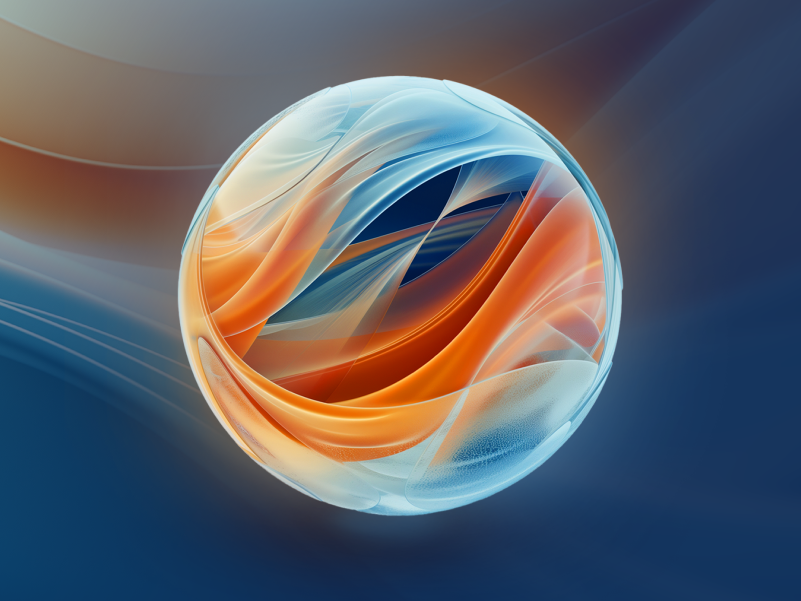
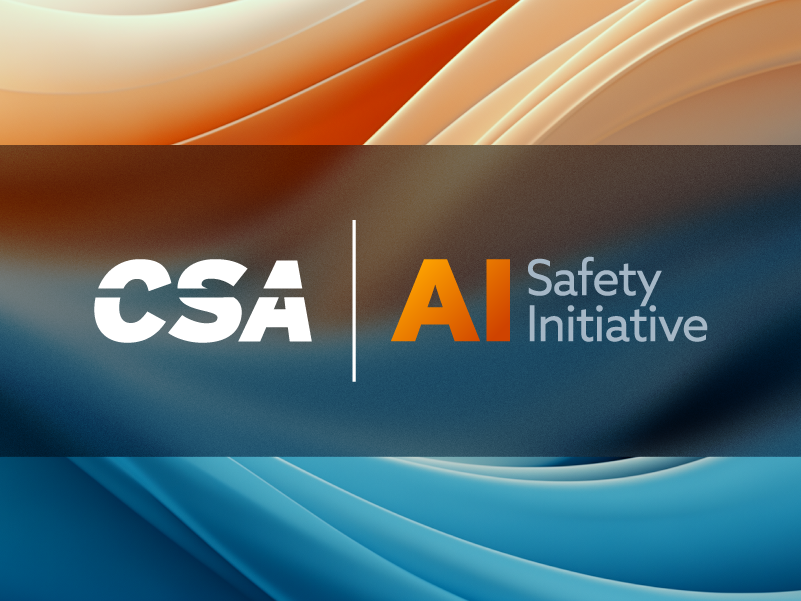
Unlock Cloud Security Insights
Subscribe to our newsletter for the latest expert trends and updates
Related Articles:
Why Identity Automation Fails at 96% of Organizations
Published: 07/07/2025
6 Key Steps to ISO 42001 Certification Explained
Published: 07/07/2025
What MITRE ATT&CK v17 Means for ESXi Security: Key Risks & How to Respond
Published: 07/03/2025
Strategic Synergy: CSA STAR, CCM, and FedRAMP 20x
Published: 07/02/2025