AI Resilience & Diversity
Published 06/20/2024
Written by Dr. Chantal Spleiss, Co-Chair of the CSA AI Governance and Compliance Working Group.
Resilience is often thrown around as a buzzword, but its true definition can be quite elusive. In this blog, I'll explore the three pillars of AI resilience: robustness, resilience, and plasticity. While robustness and resilience are vital for AI security, I'll zoom in on plasticity—an aspect that offers incredible opportunities and significant risks. Additionally, I'll highlight how incorporating diversity into AI systems can strengthen the AI landscape and offer a more resilient framework for future challenges.
Before diving into the potential triumphs and terrors, let's first understand robustness and resilience. These two pillars are foundational for AI security and trustworthiness:
- Robustness refers to an AI system's ability to withstand threats without compromising performance or changing functionality. Enhancing AI robustness requires understanding various threat vectors, which can vary significantly between simple ML applications and complex LLMs or GenAI systems. While top-notch cybersecurity is essential, each application has its own unique risks. The Cloud Security Alliance (CSA) recently published a comprehensive Threat Taxonomy for LLMs, providing valuable insights [1].
- Resilience is the capacity of an AI system to recover from incidents that impact performance, without altering the AI’s functionality. The resilience of an AI depends on the severity and frequency of impacts. As long as these two factors remain below a combined, critical threshold, the system can bounce back and return to its previous operational state. Think of resilience as a two-dimensional aspect.
Figure 1 illustrates the three pillars of AI resilience. This concept, combined with a novel benchmarking approach, is explored in the white paper “AI Resilience: A Revolutionary Benchmarking Model for AI Safety” published by the CSA during the 2024 RSA Conference [2].
Figure 1: AI Resilience
Plasticity refers to the ability of an AI to not just recover to its previous performance level after a failure but to potentially exceed it – albeit with functional changes. In humans, this ability is known as neuroplasticity, which allows the brain to form altered or new pathways to conquer daily life. When doctors speak of a "full recovery" after a brain injury, they refer to functional recovery, not a return to the original brain wiring. Similarly, plasticity in AI enables adaptation to new circumstances and learning from failures to meet or exceed performance benchmarks. Plasticity is a multi-dimensional concept.
AI Resilience: a Sharp and Double-Edged Sword
In offensive security, a field within cybersecurity, the use of AI agents is starting to gain traction. An AI agent is a software entity that perceives its environment, performs tasks, takes actions and makes decisions independently, often combined with the ability to learn and adapt over time in order to achieve specific goals. The key points of an AI agent are:
Autonomy: It operates without direct human intervention, making its own decisions and taking actions based on its programming and learned experiences.
Perception: It gathers information through sensors, data inputs, or autonomous data collection to understand its environment.
Action: It takes actions within its (specified) environment to achieve specific goals, ranging from simple commands to complex operations.
Learning and Adaptation: It learns from experiences and improves performance over time, potentially altering its code or algorithms.
Goal-Oriented Behavior: It is designed to achieve specific objectives or goals that guide its decision-making processes.
Interactivity: It interacts with other agents, humans, or systems to perform collaborative tasks or provide services.
Cybersecurity experts often stress that it's not a question of if, but when a company will be successfully attacked. Offensive security practices help prevent disastrous cyber-attacks. However, AI agents aren't just tools for cybersecurity teams – bad actors use them too, and they aren't concerned with ethics, fairness, or privacy. The havoc an AI agent can wreak with a few malicious tweaks is beyond imagination. While AI agents can protect our most valuable assets, they also have the potential to destroy them.
AI Resilience Must Be Based on Diversity
Standardizations and regulations drive automation in both the digital world and operational technology. International standards facilitate trade and disaster recovery. For instance, the standardization of hydrants was driven by the “Great Baltimore Fire” [3] in 1904, highlighting the crucial role of standards and regulations.
On the flip side, standards can create concentration risk through uniform vulnerabilities, making it easier and faster to exploit systems that adhere to them. In critical infrastructure, standards can be lifesaving—but they can also pose significant risks. So, where do we draw the line?
Nature offers a successful compromise: certain features are highly standardized, like DNA (humans and pigs share 98% of their genetic material) yet allow for adjustments through epigenetics and individual fine-tuning via selective protein expression depending on the environment.
The lesson from nature is clear – and we need to learn it quickly: standardize critical interfaces but allow innovative space for country-specific, organization-specific, or even individual solutions to achieve the same goals. Current legislation like SEC, NIS2, and DORA are all quite vaguely formulated but binding in their respective purpose. This flexibility allows for diverse and unique solutions, which is crucial. Only approaches based on diversity with varied strengths and weaknesses can provide long-term stability in our fast-evolving digital world.
Applying diversity might not be the cheapest or fastest way to tackle emerging challenges, but it’s the only approach with the potential to successfully address looming threats. This strategy also underscores the need for creative solutions beyond the beaten path.
Check out CSA’s AI Safety Initiative to learn more about safe AI usage and adoption.
References
[1]: Large Language Model (LLM) Threats Taxonomy, CSA, June 2024
[2]: AI Resilience: A Revolutionary Benchmarking Model for AI Safety, CSA, May 2024
[3]: The Great Baltimore Fire, Online Resource, Accessed: 16. June 2024.
Related Resources
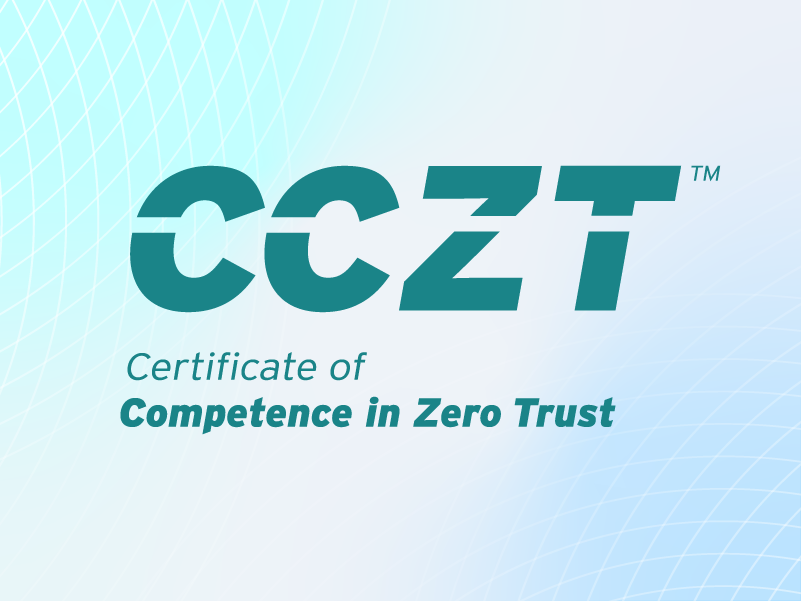
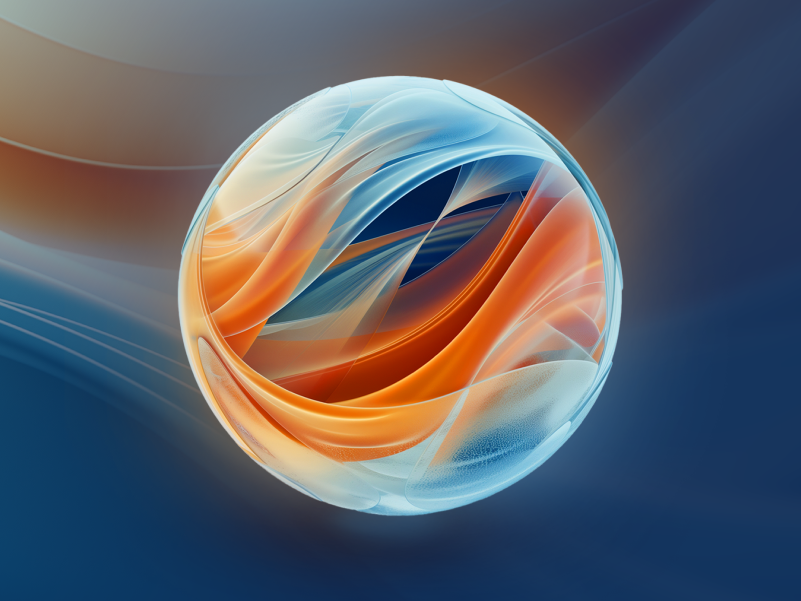
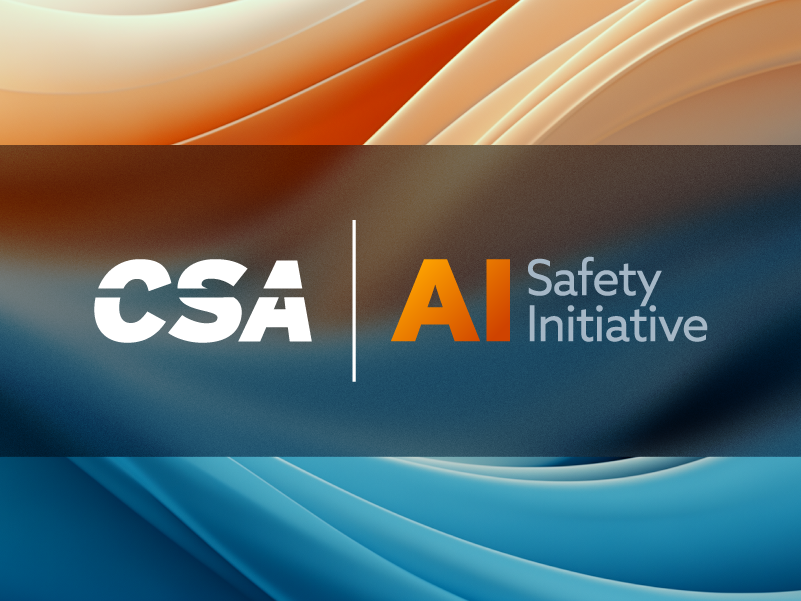
Unlock Cloud Security Insights
Subscribe to our newsletter for the latest expert trends and updates
Related Articles:
Understanding Security Risks in AI-Generated Code
Published: 07/09/2025
Why Identity Automation Fails at 96% of Organizations
Published: 07/07/2025
6 Key Steps to ISO 42001 Certification Explained
Published: 07/07/2025
The Traditional Technology Adoption Curve Doesn’t Work for AI
Published: 07/02/2025