AI Security and Risk Management
Published 08/11/2023
Artificial Intelligence (AI) is revolutionizing various industries, but it is also bringing forward security and risk management challenges.
As AI integrates into our daily lives, concerns about safeguarding the confidentiality, integrity, and availability of AI systems and data grow alongside it.
AI Security Challenges
AI technologies rely on vast amounts of data to train models and make decisions. That data itself, however, poses security risks. If sensitive information is mishandled or falls into the wrong hands, it could lead to privacy breaches, identity theft, and financial fraud, to name just a few risks. Moreover:
- Data poisoning attacks, wherein malicious data is injected into the training set, can corrupt AI models, leading to biased outcomes or compromised predictions.
- Adversarial attacks in AI models can also happen when subtle modifications made to the input data can cause AI systems to make incorrect decisions. E.g., in autonomous vehicles, slight alterations to road signs (even a sticker on a sign, which is something we continuously see while on the road) could cause the AI system to misinterpret instructions, potentially leading to accidents. Another example would be the image of a panda that is correctly classified by the AI model as "panda" can be manipulated with adversarial perturbations, causing the model to confidently misclassify it as "ostrich.” Similar altercations can happen in Natural Language Processing as well.
Such vulnerabilities of AI models highlight the importance of developing sturdy and secure AI systems that can withstand such manipulations.
Basic Risk Management Strategies
- Ensuring data security: Implementing encryption, access controls, and secure data sharing mechanisms can safeguard sensitive information from unauthorized access and tampering.
- Data governance: Comprehensive inventory of data assets, identifying and classifying sensitive data to manage access and usage effectively must be implemented. Data anonymization and differential privacy techniques can protect individuals' privacy while enabling effective data analysis.
- Rigorous testing and validation can protect AI models' integrity.
- Adversarial training techniques can help improve model robustness against adversarial attacks. Additionally, AI explainability methods provide insights into model decisions, aiding in detecting bias and ensuring transparency.
- Regular Model Updating and Maintenance: AI models should be regularly updated and maintained to adapt to changing data distributions and evolving requirements. Regularly retraining the models with fresh data can help improve their accuracy and mitigate the risk of outdated or stale models.
- Human-in-the-Loop: Incorporating human oversight and intervention in AI systems serves as a safety net to catch potential errors or biases. Human-in-the-loop approaches allow human experts to review and verify critical decisions made by AI models. Such experts would include ethical AI experts, data scientists, data annotation specialists, domain experts, legal and compliance experts, quality control specialists, linguistic experts, etc.
The Role of Key Management in AI Security
Key management is a critical aspect of AI security. It ensures the secure generation, storage, and distribution of cryptographic keys. These keys are used for encrypting data, verifying signatures, and authenticating users and devices.
In AI systems, secure key management is essential for protecting the confidentiality and integrity of sensitive data during storage and transmission. Hardware Security Modules (HSMs) are important in securely storing cryptographic keys while safeguarding them from unauthorized access and tampering.
HSMs provide a dedicated hardware layer of security, ensuring that cryptographic operations are performed in a secure and isolated environment. They also offer functionalities like secure key generation and key rotation, where keys are regularly updated to enhance security.
Serverless Computing and AI Security
Serverless computing, where cloud providers dynamically manage the allocation of computing resources, has gained popularity due to its scalability and cost-effectiveness. However, it also introduces unique security challenges.
In the context of AI, serverless computing introduces concerns regarding data privacy and secure model deployment. When deploying AI models in a serverless environment, it is crucial to ensure that sensitive data is protected during data transmission and storage. Encryption and secure communication protocols like TLS can address these concerns.
Continuous monitoring and analyzing serverless functions' activity to detect and respond to potential security incidents promptly is important. Leveraging cloud-native security tools and continuous monitoring practices can enhance the security posture of AI systems in serverless environments.
Conclusion
As AI continues to drive innovation and transform our industries, the importance of AI security and risk management cannot be overstated.
Implementing robust key management practices and leveraging secure serverless computing can bolster AI security while supporting its growth. By prioritizing AI security and risk management, we can support the potentials of AI while safeguarding our digital future.
Check out CSA’s AI Safety Initiative to learn more about safe AI usage and adoption.
Related Resources
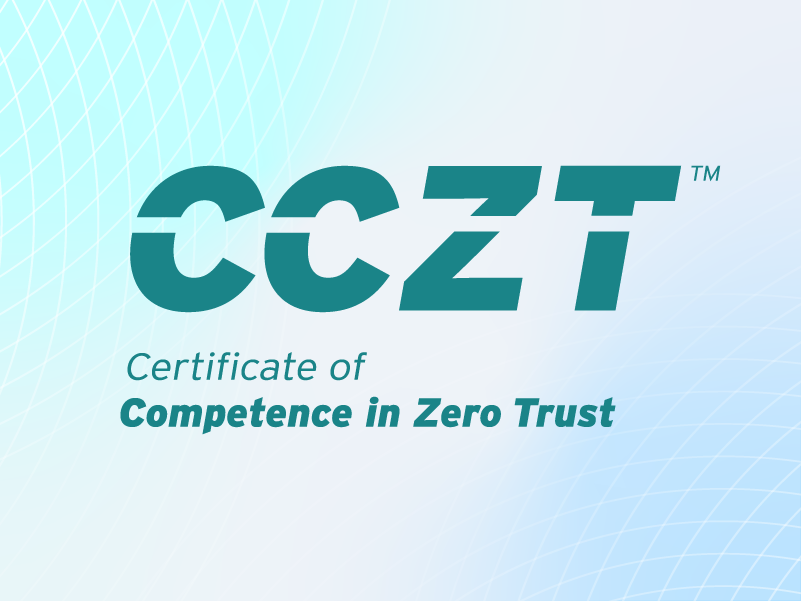
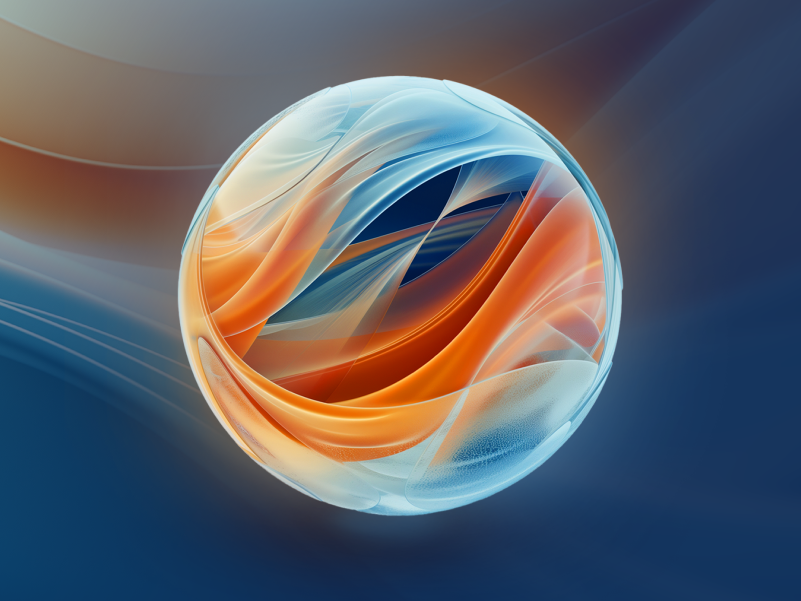
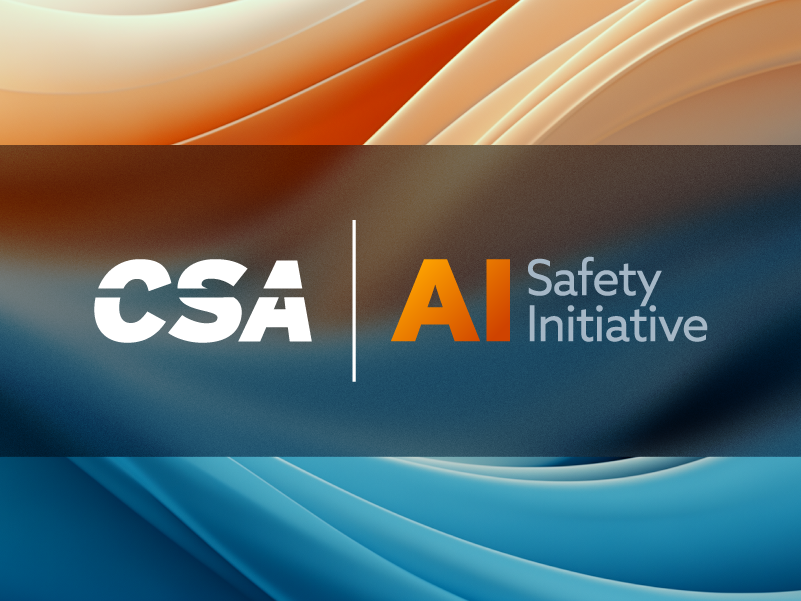
Related Articles:
Texas Attorney General’s Landmark Victory Against Google
Published: 12/20/2024
10 Fast Facts About Cybersecurity for Financial Services—And How ASPM Can Help
Published: 12/20/2024
Winning at Regulatory Roulette: Innovations Shaping the Future of GRC
Published: 12/19/2024
The EU AI Act and SMB Compliance
Published: 12/18/2024