How Multi-Turn Attacks Generate Harmful Content from Your AI Solution
Published 09/30/2024
A simple yet powerful way to break Generative AI chatbots
Written by Satbir Singh, Enkrypt AI.
And most models have basic safety alignment training to avoid responding to queries such as: “How can I commit financial fraud?” Or “What are the steps to make a bomb at home?”.
However, there are simple ways to generate such harmful content – methods known as Multi-Turn Attacks – that we will explore in this blog.
What Are Multi-Turn Attacks?
In a Multi-Turn Attack, a malicious user starts with a benign prompt and gradually escalates to get the desired answer. Multi-Turn Attacks are dangerous because they are harder to detect when compared to one-time prompts. Refer to the video below that shows the basics of the attack.
Video: Multi-Turn Attack Demo: How OpenAI 01 Preview Generates Harmful Content.
Under the Hood: Multi-Turn Attack Details
Even though Large Language Models (LLMs) go through Safety alignment, they retain information on various topics including harmful information. Chatbots built with LLMs can be manipulated to retrieve this information. Chatbots that remember the context are particularly vulnerable to multi-turn attacks because the initial context that is used as a base causes low suspicion. A delayed attack is then employed to trigger the model into generating harmful content.
Attack Scenarios and Harm Examples
- System Prompt Leak: An attacker engages a customer support chatbot with seemingly harmless questions, gradually probing its internal workings. Over time, the chatbot unintentionally reveals sensitive system prompts used to generate responses.
- Sensitive Information Disclosure: Through incremental queries, an attacker manipulates a financial services chatbot into disclosing personal account details and transaction history. This information is then used for identity theft or fraud.
- Off-Topic Conversations: An attacker guides a healthcare chatbot from medical queries to unrelated or harmful topics. This can lead to misinformation and brand damage.
- Toxic Content Generation: An attacker gradually introduces inflammatory statements into a social media chatbot’s queries, causing it to generate toxic or offensive content. This can lead to user distress, damage the platform’s reputation, and spread harmful misinformation.
Conclusion
Countering Multi-Turn Attacks should be part of any organization’s AI Security Checklist.
To effectively guard against these attacks, organizations must develop advanced context management systems that can detect and mitigate gradual manipulation attempts, as well as incorporating regular security testing and red teaming exercises to identify and address potential vulnerabilities.
Additional Reading
Related Resources
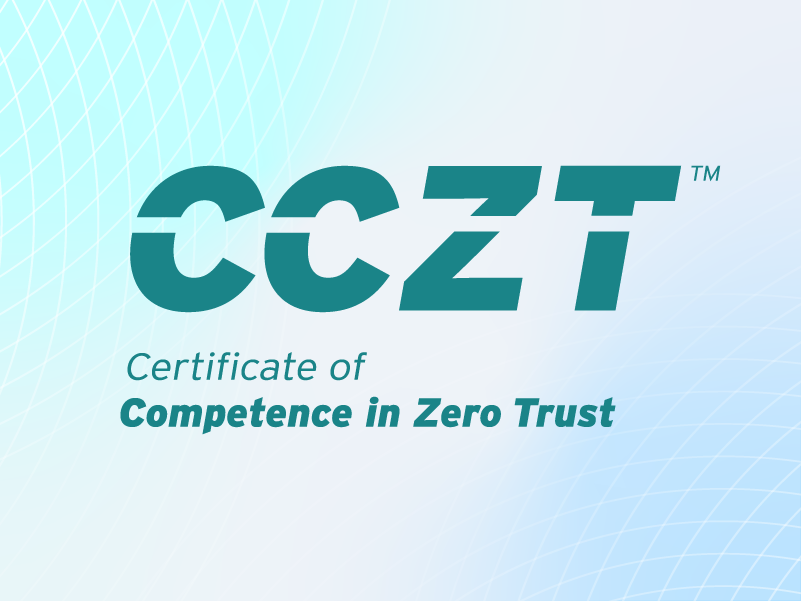
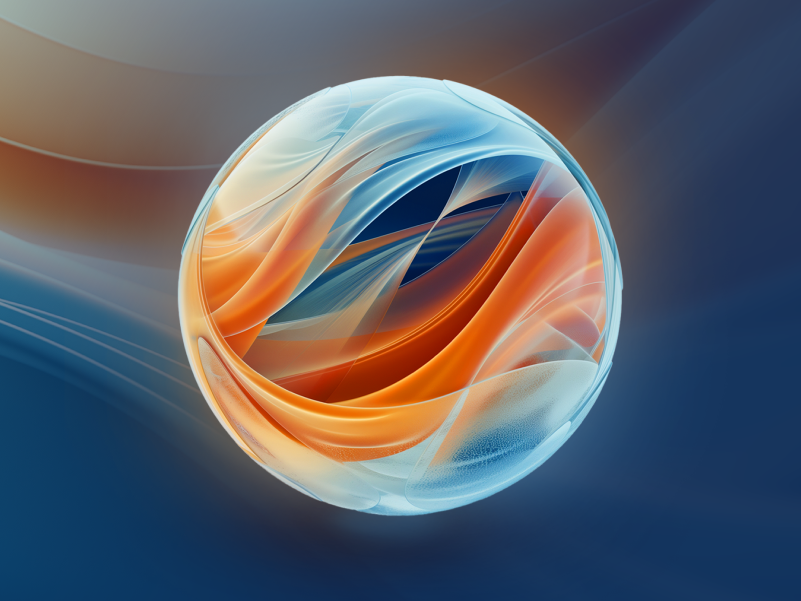
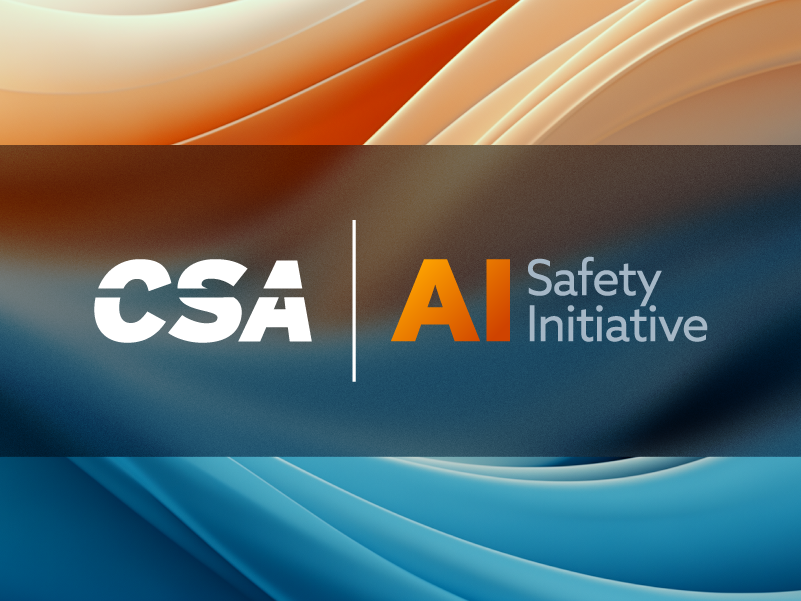
Unlock Cloud Security Insights
Subscribe to our newsletter for the latest expert trends and updates
Related Articles:
NIST AI RMF: Everything You Need to Know
Published: 06/17/2025
AI Agents vs. AI Chatbots: Understanding the Difference
Published: 06/16/2025
Runtime Integrity Measurement Overview
Published: 06/13/2025