Integrating PSO with AI: The Future of Adaptive Cybersecurity
Published 07/23/2024
Written by Sayali Paseband, Senior Security Consultant, Verisk.
In the ever-evolving landscape of cybersecurity, where threats are becoming more sophisticated, advanced, and pervasive, conventional defense mechanisms are no longer sufficient. We need to develop new technologies and innovations to stay ahead of the cyber adversaries. This blog explores an innovative solution - a novel approach that blends Particle Swarm Optimization (PSO) with artificial intelligence (AI). This combination can really transform how organizations protect themselves from cyber-attacks. This integration promises to deliver adaptive, intelligent, and proactive cybersecurity solutions that can swiftly respond to emerging risks and evolving attack vectors.
Introduction
Particle Swarm Optimization (PSO) is an effective optimization method that uses the social behavior of swarms (groups of living beings like ants or bees) as a model. It continuously improves solutions using the knowledge acquired by each member of a swarm. In cybersecurity, PSO algorithms can optimize security parameters and policies dynamically, enhancing threat detection, and response capabilities with minimal human intervention.
AI, on the other hand, empowers cybersecurity systems with the ability to learn from data, recognize patterns, and make decisions autonomously. By integrating AI algorithms like machine learning and deep learning with PSO, cybersecurity measures can adapt in real-time to changing threats, predict future attacks, and optimize defenses proactively.
Advantages of Integration
1. Dynamic Threat Detection
AI-enhanced PSO algorithms significantly enhance the capability to detect and respond to emerging threats in real-time. Here's how:
Anomaly Detection: AI algorithms, such as machine learning models, are trained on large datasets to recognize patterns of normal and abnormal behavior in network traffic and system activities. Within PSO frameworks, these algorithms continuously analyze incoming data streams. When anomalies are detected, PSO adjusts parameters dynamically, such as thresholds for alert triggers or rules for anomaly classification.
Behavioral Analysis: AI-powered PSO uses behavioral analysis to identify suspicious patterns that may indicate new or evolving threats. For example, AI can detect deviations from established user behavior or network traffic patterns that suggest potential security breaches.
Automated Response: Once a threat is identified, AI-driven PSO can initiate automated responses. This includes actions like isolating compromised systems, blocking suspicious IP addresses, or dynamically updating firewall rules. AI algorithms make decisions based on predefined policies and real-time threat intelligence, ensuring swift and effective mitigation of security incidents.
2. Optimized Security Policies
The integration of PSO with AI facilitates the dynamic optimization of security policies based on evolving threats and system conditions:
Continuous Learning: AI algorithms integrated with PSO frameworks continuously learn from new data and feedback loops. This learning process allows them to adapt security policies dynamically in response to changing threat landscapes and operational conditions. For instance, AI can analyze historical attack patterns to predict future threats and adjust security configurations preemptively.
Real-Time Adjustments: AI-powered PSO enables real-time adjustments of security parameters based on current threat intelligence and network dynamics. This agility ensures that security measures are always aligned with the most current and relevant information, minimizing the window of vulnerability to emerging threats.
3. Scalability and Efficiency
Integrating AI with PSO enhances scalability and operational efficiency in cybersecurity:
Resource Optimization: AI algorithms optimize the allocation of computational resources within PSO frameworks, ensuring efficient processing of security data and analytics. This optimization minimizes computational overhead and improves the responsiveness of cybersecurity operations. For example, AI can prioritize the processing of critical security alerts or optimize the allocation of computing resources for intensive tasks like deep packet inspection or malware analysis.
Management of Complexity: In heterogeneous or complex IT environments, AI-driven PSO algorithms streamline the management of complex security protocols and configurations. AI algorithms automate routine tasks such as policy updates, vulnerability assessments, and incident response coordination, reducing the burden on cybersecurity teams and ensuring consistent adherence to security policies.
Performance Enhancement: The integration improves the overall performance of cybersecurity systems by leveraging AI's capabilities in pattern recognition, predictive analytics, and adaptive learning. This enhancement leads to faster threat detection, more accurate risk assessments, and timely incident response actions.
Conclusion
The integration of PSO with AI marks a significant leap forward in cybersecurity, offering organizations adaptive, intelligent, and scalable defenses against the evolving threat landscape. By leveraging the collective intelligence of optimization algorithms and the learning capabilities of artificial intelligence, businesses can fortify their cybersecurity posture, mitigate risks effectively, and safeguard the most critical assets. This integration not only strengthens cybersecurity posture but also enables proactive defense strategies against modern, evolving cyber threats, thereby maintaining resilience in dynamic digital environments.
Related Resources
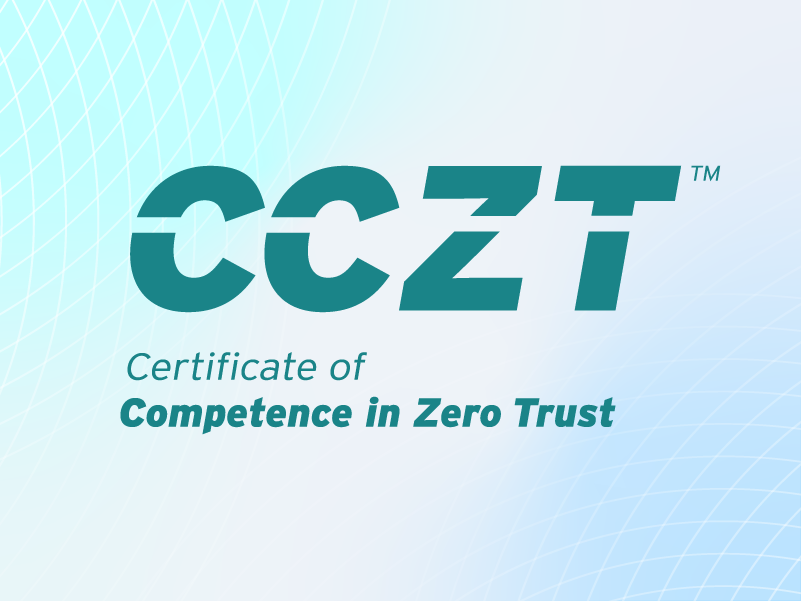
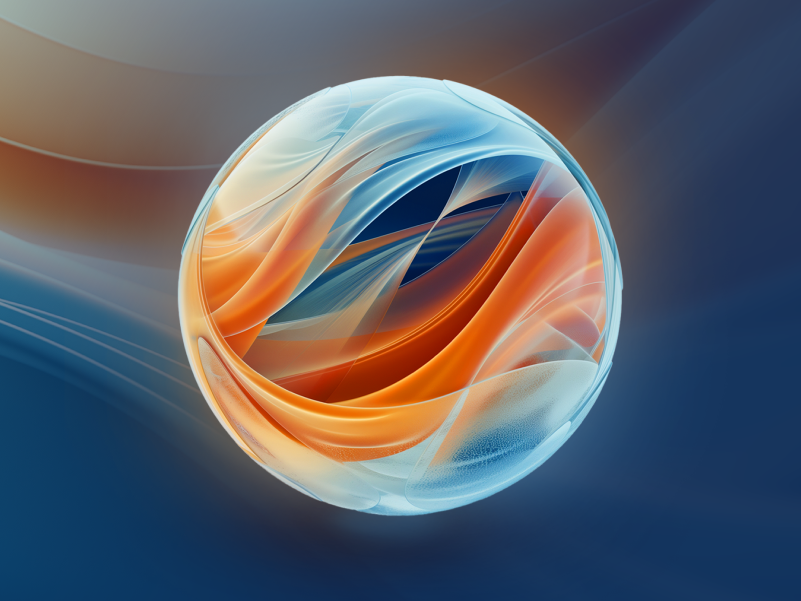
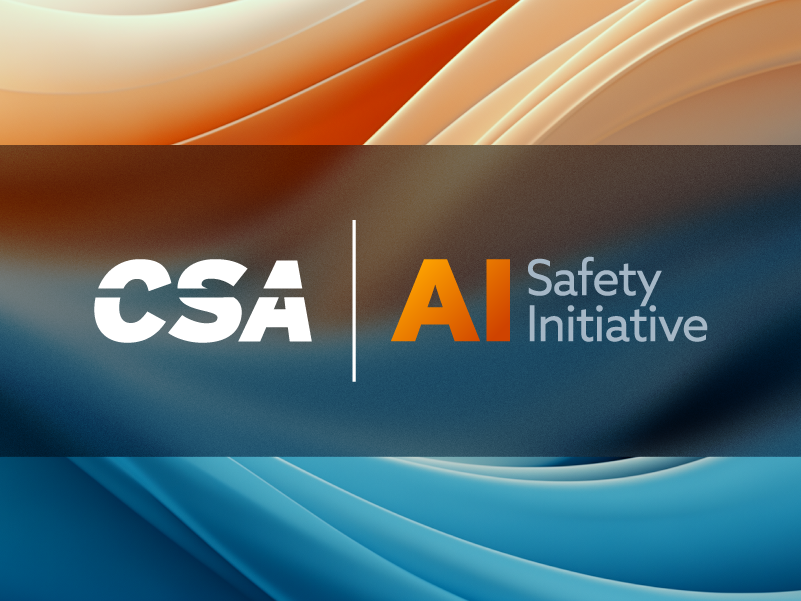
Related Articles:
Texas Attorney General’s Landmark Victory Against Google
Published: 12/20/2024
10 Fast Facts About Cybersecurity for Financial Services—And How ASPM Can Help
Published: 12/20/2024
Winning at Regulatory Roulette: Innovations Shaping the Future of GRC
Published: 12/19/2024
The EU AI Act and SMB Compliance
Published: 12/18/2024