Into the Abyss: How a Dark Web LLM Could Enhance Our Cybersecurity
Published 08/28/2023
Originally published by CXO REvolutionaries.
Written by Kyle Fiehler, Senior Transformation Analyst, Zscaler.
Amid nuanced debate about whether AI will save the world or rise to kill us all, why train a large language model (LLM) on roughly 6.1 million pages of dark web content?
Research, says one group of South Korean academics in a paper titled DarkBERT: A Language Model for the Dark Side of the Internet published in May.
The dark web has long been a valuable source of study for academic researchers and security practitioners alike. Unlike a drug bazaar or a weapons dealer’s back room, the dark web is a criminal underground that can be observed from a place of relative safety, offering the potential for serious study absent the risk of serious harm.
"The continued exploitation of the Dark Web as a platform of cybercrime makes it a valuable and necessary domain for [cyber threat intelligence] research," the authors write.
As a source of cyber threat intelligence, though, it speaks its own dialect.
Due to its “lexical differences” (read: the way things are said), the researchers hypothesized that LLMs trained on the “vanilla counterpart” of the dark web (the surface web), can not possibly be well-suited to divining the same insights as a more streetwise LLM.
The model trained to test their hypothesis was dubbed DarkBERT – as it relies on the BERT framework for natural language processing (NLP) – and their findings could assist cybersecurity practitioners in classifying ransomware leak sites, identifying the release of sensitive information, and more accurately detecting dangerous posts based on keyword inferences.
Meet DarkBERT
An LLM trained on the most unsavory of online content could be a useful tool for threat researchers. Why? Remember, LLMs work by ingesting huge amounts of text, analyzing it for patterns, and predicting which words should come next. While a model trained on the entirety of Wikipedia may not be adept at spotting a social security number, credit card information, or CVE ID, these are exactly the types of information DarkBERT was trained to pinpoint.
This allows DarkBERT to perform better at spotting confidential information likely being leaked in hacker forums following a data breach. By comparing DarkBERT to its more reputable counterparts, the researchers found that their LLM was better suited to performing tasks such as page classification and activity identification than surface-web-trained alternatives.
Exploring cybersecurity use cases
The paper’s most exciting section explores how DarkBERT’s superior performance on the dark web could be useful to threat researchers and other security professionals.
It explores three main use cases:
1. Detecting ransomware leak sites
Ransomware actors typically take to dark websites to either:
- Expose their victims and further threaten to leak their data (often accompanied by a small data sample as proof of compromise);
-OR-
- To publish data stolen from uncooperative victims to coerce payment for the balance of misappropriated data.
Given this tendency, the paper’s authors reasoned that an LLM that can more accurately identify such leak sites would be useful to threat researchers looking to understand the actions of ransomware groups. DarkBERT did just that.
The researchers monitored leak sites belonging to 54 ransomware groups for two years. They found that their model was able to more accurately identify these ransomware leak sites than surface-web-trained models.
2. Identifying “noteworthy” threads
From the authors’ standpoint, this is an admittedly subjective task. Still, they defined noteworthy threads as "activities in hacking forums that can potentially cause damage to a wide range of victims," and enlisting the help of professional threat researchers, the authors settled on attempting to recognize threads containing:
- Sensitive organizational information, including admin access credentials, employee information, transaction histories, blueprints, source code, and other documents likely to be considered confidential
- Sensitive information belonging to individuals, including credit card numbers, medical records, histories of political involvement, passport numbers, etc.
- Attempts to distribute malware or vulnerabilities targeting specific organizations.
Once again, DarkBERT was more successful in identifying these threats than surface-web-trained models, scoring higher in precision, recall, and F1 scores. (An F1 score is a combination of precision and recall scores.) This capability could help threat researchers locate the source of stolen data more quickly and notify victims of its compromise.
3. Threat keyword inference
In the third use case, the researchers focused on using the fill-mask function – hiding a word and prompting the natural language processing (NLP) engine to guess what it may be – in order to generate a list of keywords that may be involved in illicit activities like drug sales.
When asked, for example, for a list of related words that might follow “Dutch” on an ad for an illegal drug sold by a Netherlands-based user, a surface-web-trained model made guesses like “champion,” “sculptor,” “citizen,” and other “vocational” words one might expect Dutch to modify on the legitimate internet. DarkBERT, on the other hand, did a much better job of specifying a list of words one might expect to be associated with a drug-related transaction, such as “pills” and “speed.” The researchers reason that this ability will be useful in recognizing advertisements for drug sales even when they feature slang terms not widely used on the surface web.
Possible practical applications?
It’s neither the researchers’ aim nor their responsibility to work through how cybersecurity practitioners could best use these capabilities. Like most AI applications, a DarkBERT-style bot could also have malicious applications; it could act as a dark web personal shopping assistant, helping cybercriminals quickly find the stolen information they’re looking for.
But it’s also clear that dark-web-trained LLMs could help security researchers with the right skills to trawl the more unsavory corners of the internet for the most egregious threats like weapons sales and crimes involving minors. Given all of the dubious applications LLMs will undoubtedly be deployed for, it’s nice to see another way they can undermine dark web threat actors.
Luckily, the paper’s authors pledge to keep DarkBERT’s eyes on the dark web. And to keep us posted on what it learns.
Related Resources
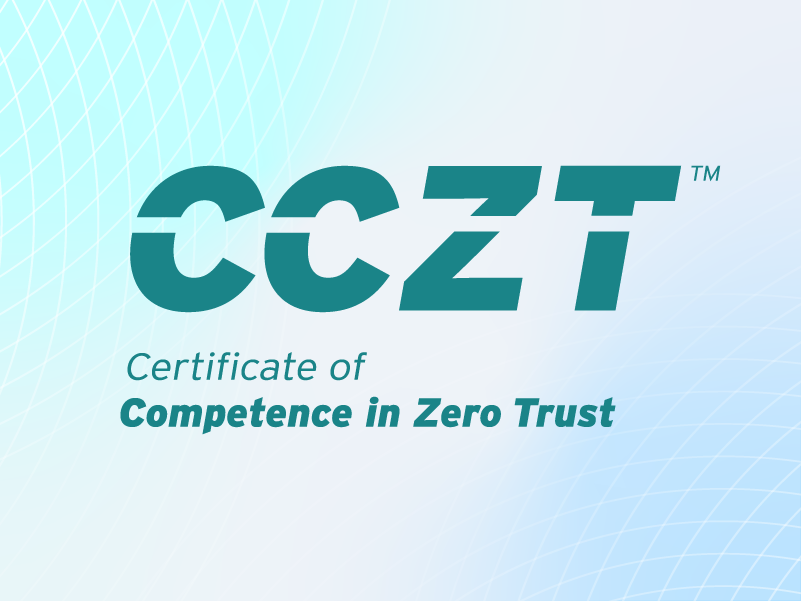
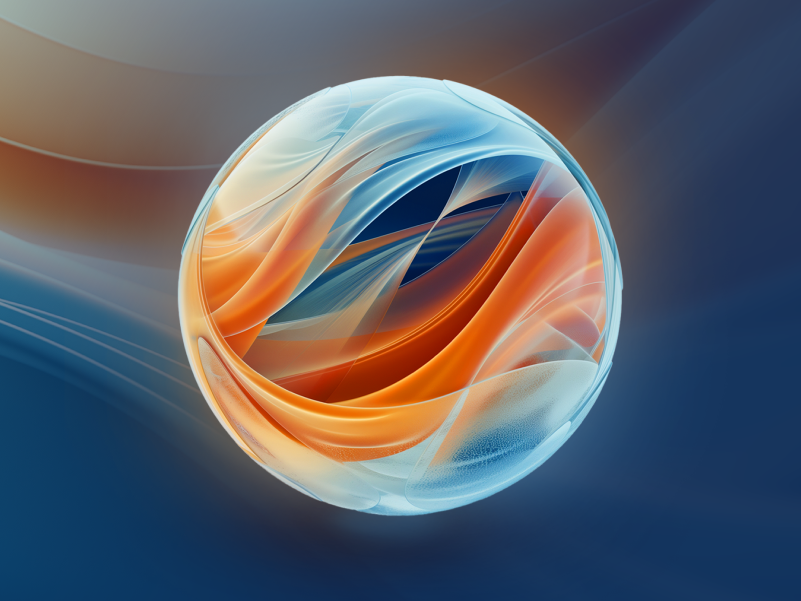
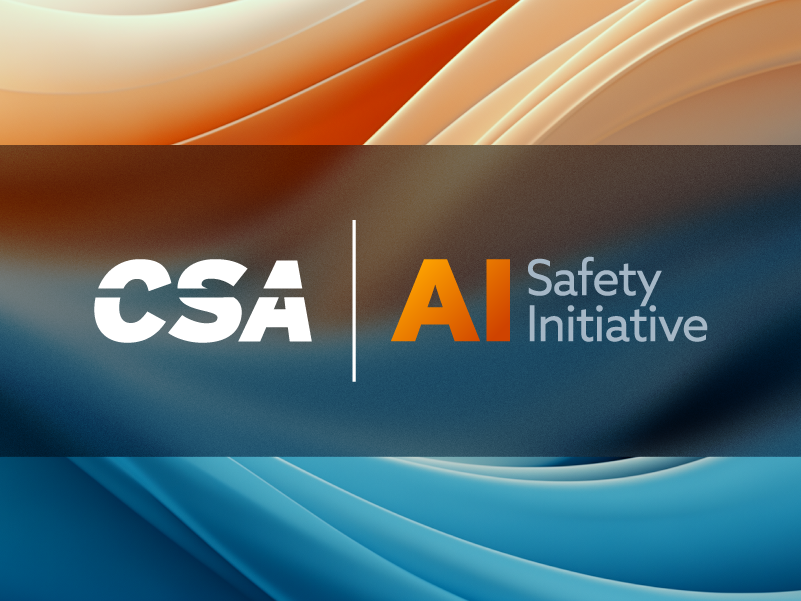
Related Articles:
CSA Community Spotlight: Nerding Out About Security with CISO Alexander Getsin
Published: 11/21/2024
Establishing an Always-Ready State with Continuous Controls Monitoring
Published: 11/21/2024
AI-Powered Cybersecurity: Safeguarding the Media Industry
Published: 11/20/2024
5 Big Cybersecurity Laws You Need to Know About Ahead of 2025
Published: 11/20/2024