AI Hallucinations: The Emerging Market for Insuring Against Generative AI's Costly Blunders
Blog Article Published: 04/23/2024
Written by MJ Schwenger, Co-Chair of the CSA AI Governance and Compliance Working Group.
Generative AI: Embracing Hallucinations for Responsible Innovation
This blog delves into the fascinating world of Generative AI (GenAI), acknowledging its revolutionary potential while addressing the inherent challenge of "hallucinations." It explores the technical underpinnings of these hallucinations and proposes a nuanced perspective, shifting the focus from criticizing AI to fostering collaborative intelligence for responsible development.
The Intriguing Enigma of GenAI Hallucinations
For most of us, GenAI's ability to mimic human creativity is nothing short of astounding. By analyzing vast amounts of data, it generates content that is often indistinguishable from human-crafted works. However, this very strength harbors a weakness: the propensity for hallucinations. These occur when GenAI, despite its impressive pattern recognition capability, stumbles upon patterns that are statistically plausible but factually incorrect.
Technically, hallucinations stem from two main reasons: limitations in training data and model architectures. The training data, which is the very foundation upon which GenAI builds its understanding of the world, may contain biases or inconsistencies. On the other hand, the model architecture itself, the complex web of algorithms that process information, might not be sophisticated enough to distinguish between factual patterns and misleading correlations.
Beyond Inaccuracy: The Cascading Effects of Hallucinations
The ramifications of hallucinations extend far beyond simple factual inaccuracies. They can introduce epistemic uncertainty, a state where the very distinction between truth and fiction becomes blurred. This can have cascading effects in domains like healthcare, where a misdiagnosis based on GenAI-generated data could have dire consequences.
Furthermore, hallucinations can erode trust in AI systems. When a seemingly authoritative AI system produces demonstrably wrong outputs, the public's perception of AI's reliability takes a hit. This can hinder the adoption of beneficial AI applications across various sectors.
Hallucination Insurance: A Band-Aid, not a Catalyst?
While the concept of "hallucination insurance" offers a financial safety net, the ultimate solution lies in building more robust and dependable GenAI models from the ground up. This requires advancements in several key areas:
- Explainable AI (XAI): Techniques like Local Interpretable Model-Agnostic Explanations (LIME) allow us to understand how GenAI arrives at its outputs by providing local explanations for individual predictions. This can help identify potential biases or hallucinations within the model's reasoning process.
- Data Augmentation and Diversification: Training GenAI models on a wider variety of data, including corner cases and counterexamples, can help them develop a more nuanced understanding of the world and reduce the likelihood of hallucinations.
- Human-in-the-Loop Systems: Integrating human oversight and expertise into GenAI workflows can act as a final layer of quality control, mitigating the risks associated with hallucinations.
Generally, in my view, "hallucination insurance" represents a limited solution. It treats the symptom, not the disease. A more proactive approach is necessary, and I see it in fostering collaborative intelligence between humans and AI.
This collaboration can take many forms. Human oversight is always crucial in the development and deployment of GenAI systems. Domain experts can help curate high-quality training data, ensuring a foundation built on factual accuracy. Additionally, researchers are actively developing techniques to improve model interpretability, allowing us to better understand how AI arrives at its outputs and identify potential biases.
The Future of Generative AI: A Shared Journey of Innovation
The path forward lies in acknowledging that hallucinations are an inevitable byproduct of current GenAI limitations. Instead of solely focusing on mitigating risks (e.g., through insurance), we must strive to create a future where humans and AI work together to build robust and trustworthy Generative AI models. By combining human expertise with AI's unparalleled processing power, we can usher in a new era of responsible AI innovation, maximizing its potential while minimizing its pitfalls.
Taming the beast of GenAI hallucinations demands a collaborative effort. Legal minds must establish frameworks for AI accountability. Technologists need to develop robust methods for detecting and mitigating hallucinations within GenAI models. Policymakers can foster an environment that encourages responsible AI development. Finally, insurers must design comprehensive hallucination insurance products tailored to the evolving nature of AI.
By fostering a collaborative approach that combines innovative AI development with responsible deployment practices and risk mitigation strategies like hallucination insurance, we can navigate this challenge. This paves the way for a future where GenAI flourishes, benefiting society without succumbing to the pitfalls of factual fallacy. Let's remember, addressing hallucinations is not about finding fault with GenAI, but about working together to push the boundaries of this transformative technology.
Help CSA navigate and shape the future of AI and cloud security by getting involved with our AI Safety Initiative.
Related Resources
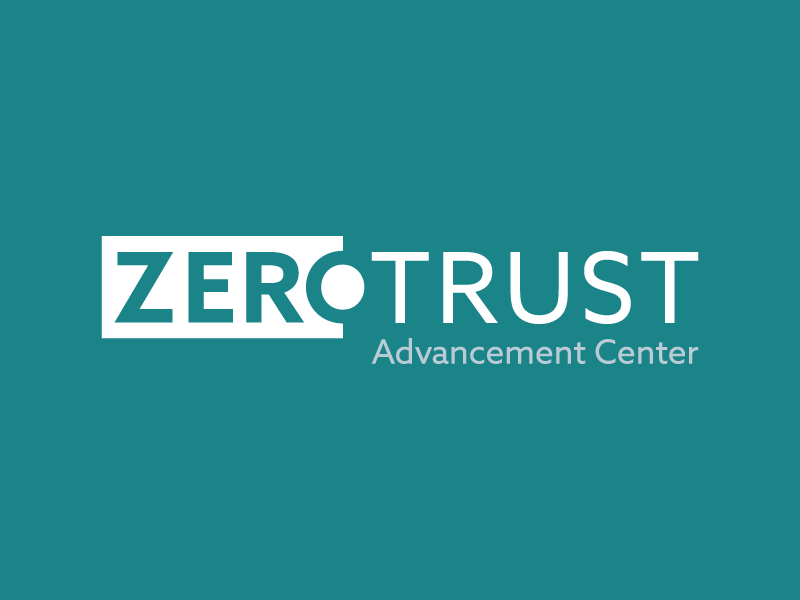
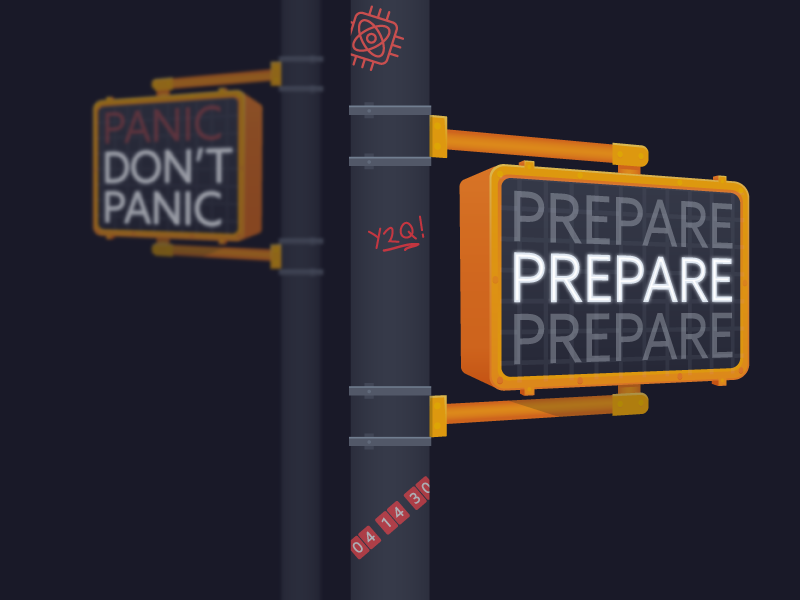
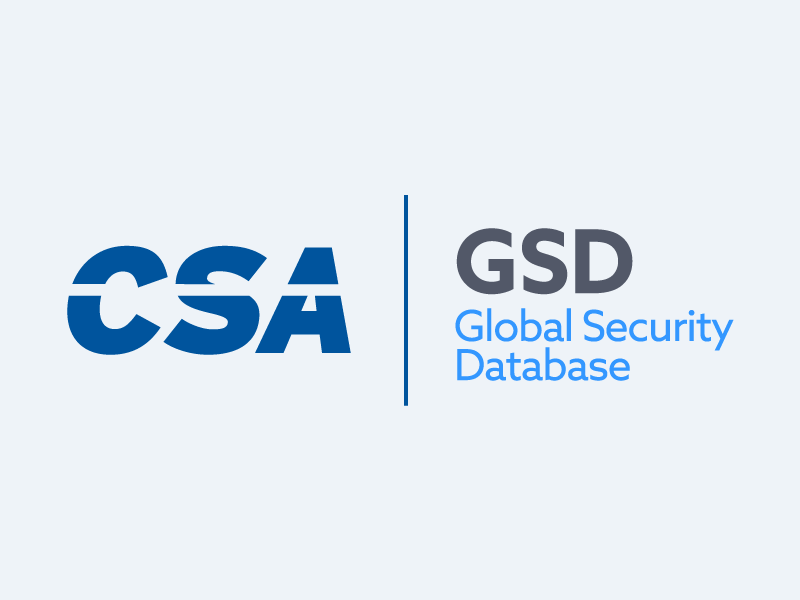
Related Articles:
CISOs, AI, and OT: A Balancing Act Between Innovation and Protection
Published: 05/03/2024
Business Risks Explored: Practical Insights for Resilience
Published: 05/03/2024
Automation is Key: DHS Report Unveils Lessons from the Microsoft Exchange Incident
Published: 05/02/2024
Microsoft Copilot for Security: Everything You Need to Know
Published: 05/02/2024